arXiv:2501.05686v1 Announce Type: new
Abstract: Cross-modal retrieval (CMR) typically involves learning common representations to directly measure similarities between multimodal samples. Most existing CMR methods commonly assume multimodal samples in pairs and employ joint training to learn common representations, limiting the flexibility of CMR. Although some methods adopt independent training strategies for each modality to improve flexibility in CMR, they utilize the randomly initialized orthogonal matrices to guide representation learning, which is suboptimal since they assume inter-class samples are independent of each other, limiting the potential of semantic alignments between sample representations and ground-truth labels. To address these issues, we propose a novel method termed Deep Reversible Consistency Learning (DRCL) for cross-modal retrieval. DRCL includes two core modules, ie Selective Prior Learning (SPL) and Reversible Semantic Consistency learning (RSC). More specifically, SPL first learns a transformation weight matrix on each modality and selects the best one based on the quality score as the Prior, which greatly avoids blind selection of priors learned from low-quality modalities. Then, RSC employs a Modality-invariant Representation Recasting mechanism (MRR) to recast the potential modality-invariant representations from sample semantic labels by the generalized inverse matrix of the prior. Since labels are devoid of modal-specific information, we utilize the recast features to guide the representation learning, thus maintaining semantic consistency to the fullest extent possible. In addition, a feature augmentation mechanism (FA) is introduced in RSC to encourage the model to learn over a wider data distribution for diversity. Finally, extensive experiments conducted on five widely used datasets and comparisons with 15 state-of-the-art baselines demonstrate the effectiveness and superiority of our DRCL.
Source link
lol
Deep Reversible Consistency Learning for Cross-modal Retrieval
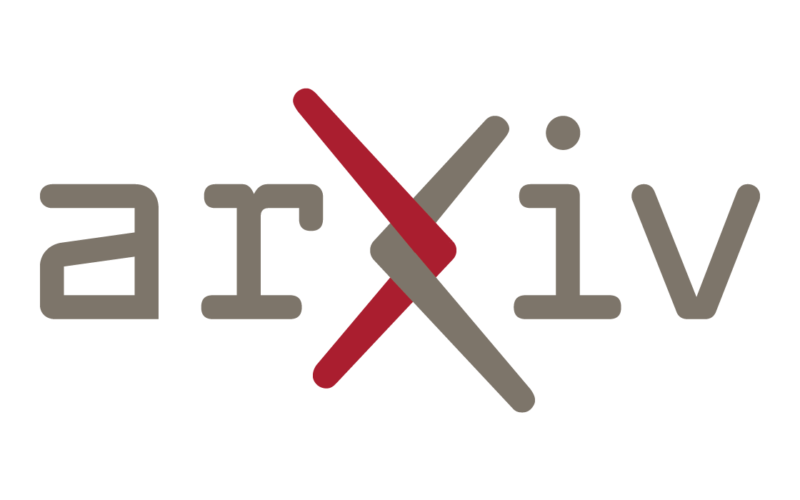