arXiv:2501.06225v1 Announce Type: new
Abstract: Medical images are characterized by intricate and complex features, requiring interpretation by physicians with medical knowledge and experience. Classical neural networks can reduce the workload of physicians, but can only handle these complex features to a limited extent. Theoretically, quantum computing can explore a broader parameter space with fewer parameters, but it is currently limited by the constraints of quantum hardware.Considering these factors, we propose a distributed hybrid quantum convolutional neural network based on quantum circuit splitting. This model leverages the advantages of quantum computing to effectively capture the complex features of medical images, enabling efficient classification even in resource-constrained environments. Our model employs a quantum convolutional neural network (QCNN) to extract high-dimensional features from medical images, thereby enhancing the model’s expressive capability.By integrating distributed techniques based on quantum circuit splitting, the 8-qubit QCNN can be reconstructed using only 5 qubits.Experimental results demonstrate that our model achieves strong performance across 3 datasets for both binary and multiclass classification tasks. Furthermore, compared to recent technologies, our model achieves superior performance with fewer parameters, and experimental results validate the effectiveness of our model.
Source link
lol
A Distributed Hybrid Quantum Convolutional Neural Network for Medical Image Classification
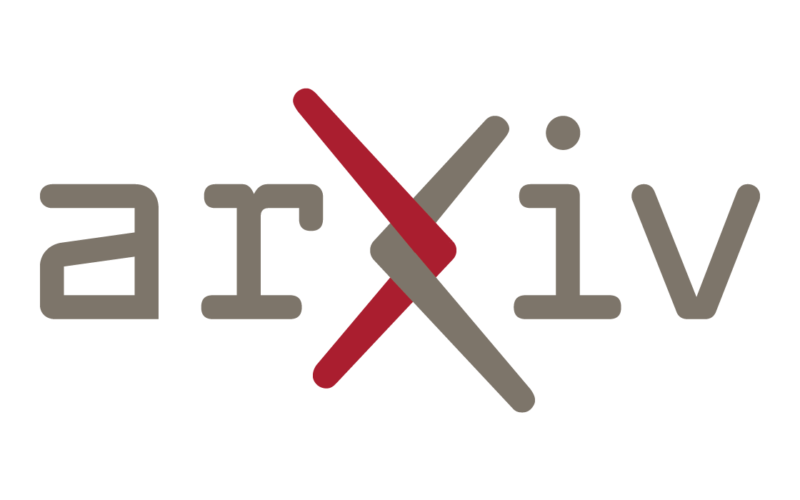