arXiv:2501.06236v1 Announce Type: new
Abstract: Modeling radio propagation is essential for wireless network design and performance optimization. Traditional methods rely on physics models of radio propagation, which can be inaccurate or inflexible. In this work, we propose using graph neural networks to learn radio propagation behaviors directly from real-world network data. Our approach converts the radio propagation environment into a graph representation, with nodes corresponding to locations and edges representing spatial and ray-tracing relationships between locations. The graph is generated by converting images of the environment into a graph structure, with specific relationships between nodes. The model is trained on this graph representation, using sensor measurements as target data.
We demonstrate that the graph neural network, which learns to predict radio propagation directly from data, achieves competitive performance compared to traditional heuristic models. This data-driven approach outperforms classic numerical solvers in terms of both speed and accuracy. To the best of our knowledge, we are the first to apply graph neural networks to real-world radio propagation data to generate coverage maps, enabling generative models of signal propagation with point measurements only.
Source link
lol
Data-Driven Radio Propagation Modeling using Graph Neural Networks
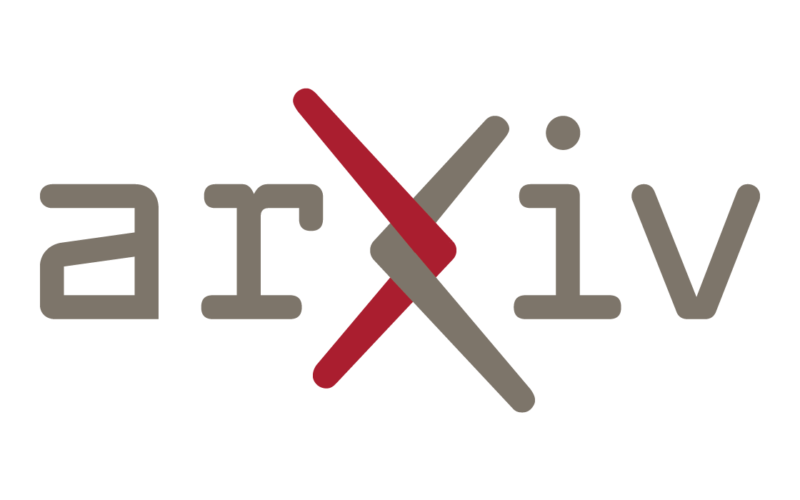