arXiv:2501.11923v1 Announce Type: cross
Abstract: In recent years, the integration of deep learning techniques with remote sensing technology has revolutionized the way natural hazards, such as floods, are monitored and managed. However, existing methods for flood segmentation using remote sensing data often overlook the utility of correlative features among multispectral satellite information. In this study, we introduce a progressive cross attention network (ProCANet), a deep learning model that progressively applies both self- and cross-attention mechanisms to multispectral features, generating optimal feature combinations for flood segmentation. The proposed model was compared with state-of-the-art approaches using Sen1Floods11 dataset and our bespoke flood data generated for the Citarum River basin, Indonesia. Our model demonstrated superior performance with the highest Intersection over Union (IoU) score of 0.815. Our results in this study, coupled with the ablation assessment comparing scenarios with and without attention across various modalities, opens a promising path for enhancing the accuracy of flood analysis using remote sensing technology.
Source link
lol
Progressive Cross Attention Network for Flood Segmentation using Multispectral Satellite Imagery
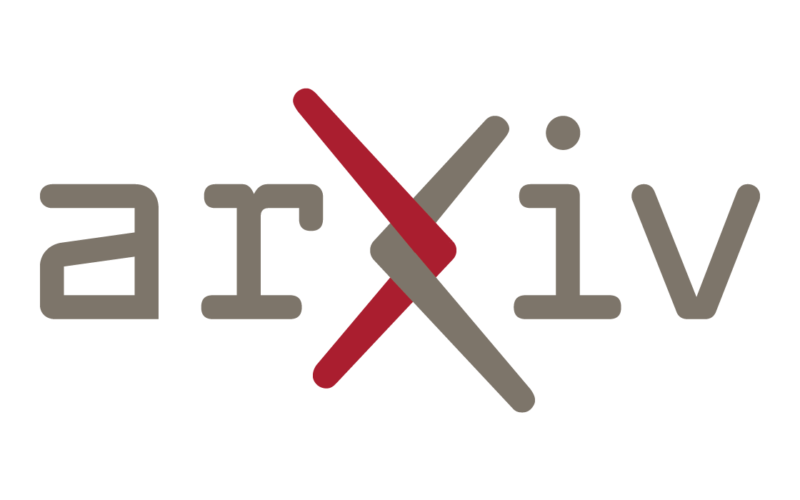