arXiv:2501.10615v1 Announce Type: new
Abstract: Accurate segmentation of the aorta and its associated arch branches is crucial for diagnosing aortic diseases. While deep learning techniques have significantly improved aorta segmentation, they remain challenging due to the intricate multiscale structure and the complexity of the surrounding tissues. This paper presents a novel approach for enhancing aorta segmentation using a Bayesian neural network-based hierarchical Laplacian of Gaussian (LoG) model. Our model consists of a 3D U-Net stream and a hierarchical LoG stream: the former provides an initial aorta segmentation, and the latter enhances blood vessel detection across varying scales by learning suitable LoG kernels, enabling self-adaptive handling of different parts of the aorta vessels with significant scale differences. We employ a Bayesian method to parameterize the LoG stream and provide confidence intervals for the segmentation results, ensuring robustness and reliability of the prediction for vascular medical image analysts. Experimental results show that our model can accurately segment main and supra-aortic vessels, yielding at least a 3% gain in the Dice coefficient over state-of-the-art methods across multiple volumes drawn from two aorta datasets, and can provide reliable confidence intervals for different parts of the aorta. The code is available at https://github.com/adlsn/LoGBNet.
Source link
lol
Hierarchical LoG Bayesian Neural Network for Enhanced Aorta Segmentation
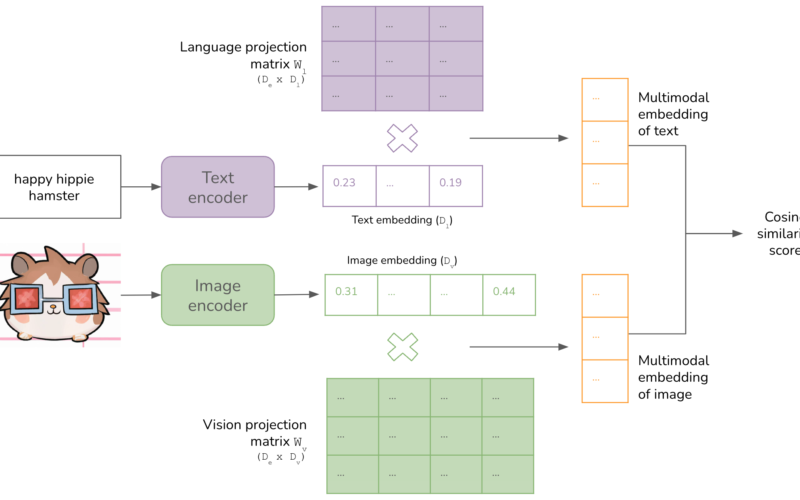