arXiv:2501.05744v1 Announce Type: new
Abstract: Video restoration plays a pivotal role in revitalizing degraded video content by rectifying imperfections caused by various degradations introduced during capturing (sensor noise, motion blur, etc.), saving/sharing (compression, resizing, etc.) and editing. This paper introduces a novel algorithm designed for scenarios where noise is introduced during video capture, aiming to enhance the visual quality of videos by reducing unwanted noise artifacts. We propose the Latent space LSTM Video Denoiser (LLVD), an end-to-end blind denoising model. LLVD uniquely combines spatial and temporal feature extraction, employing Long Short Term Memory (LSTM) within the encoded feature domain. This integration of LSTM layers is crucial for maintaining continuity and minimizing flicker in the restored video. Moreover, processing frames in the encoded feature domain significantly reduces computations, resulting in a very lightweight architecture. LLVD’s blind nature makes it versatile for real, in-the-wild denoising scenarios where prior information about noise characteristics is not available. Experiments reveal that LLVD demonstrates excellent performance for both synthetic and captured noise. Specifically, LLVD surpasses the current State-Of-The-Art (SOTA) in RAW denoising by 0.3dB, while also achieving a 59% reduction in computational complexity.
Source link
lol
LLVD: LSTM-based Explicit Motion Modeling in Latent Space for Blind Video Denoising
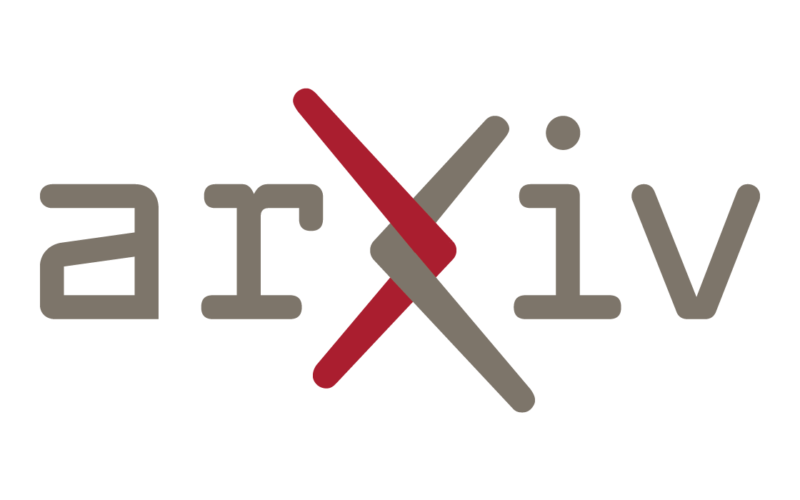