arXiv:2501.09967v1 Announce Type: new
Abstract: Artificial Intelligence (AI) has continued to achieve tremendous success in recent times. However, the decision logic of these frameworks is often not transparent, making it difficult for stakeholders to understand, interpret or explain their behavior. This limitation hinders trust in machine learning systems and causes a general reluctance towards their adoption in practical applications, particularly in mission-critical domains like healthcare and autonomous driving. Explainable AI (XAI) techniques facilitate the explainability or interpretability of machine learning models, enabling users to discern the basis of the decision and possibly avert undesirable behavior. This comprehensive survey details the advancements of explainable AI methods, from inherently interpretable models to modern approaches for achieving interpretability of various black box models, including large language models (LLMs). Additionally, we review explainable AI techniques that leverage LLM and vision-language model (VLM) frameworks to automate or improve the explainability of other machine learning models. The use of LLM and VLM as interpretability methods particularly enables high-level, semantically meaningful explanations of model decisions and behavior. Throughout the paper, we highlight the scientific principles, strengths and weaknesses of state-of-the-art methods and outline different areas of improvement. Where appropriate, we also present qualitative and quantitative comparison results of various methods to show how they compare. Finally, we discuss the key challenges of XAI and directions for future research.
Source link
lol
Explainable artificial intelligence (XAI): from inherent explainability to large language models
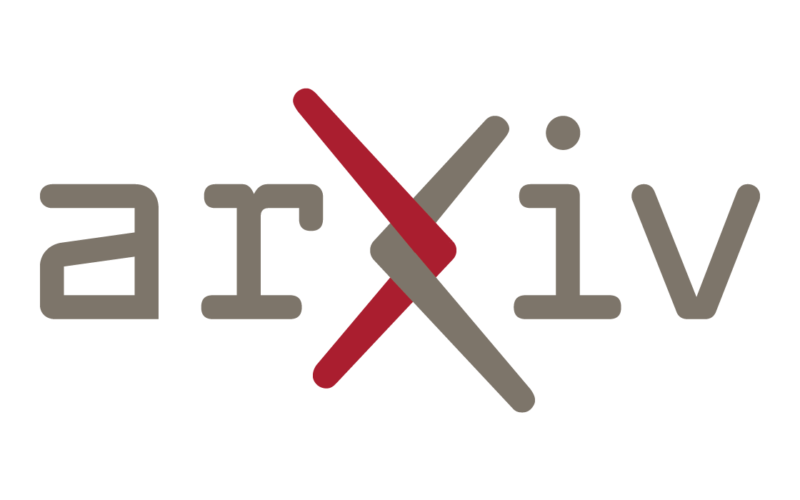