arXiv:2501.04970v1 Announce Type: new
Abstract: Deep Neural Networks have spearheaded remarkable advancements in time series forecasting (TSF), one of the major tasks in time series modeling. Nonetheless, the non-stationarity of time series undermines the reliability of pre-trained source time series forecasters in mission-critical deployment settings. In this study, we introduce a pioneering test-time adaptation framework tailored for TSF (TSF-TTA). TAFAS, the proposed approach to TSF-TTA, flexibly adapts source forecasters to continuously shifting test distributions while preserving the core semantic information learned during pre-training. The novel utilization of partially-observed ground truth and gated calibration module enables proactive, robust, and model-agnostic adaptation of source forecasters. Experiments on diverse benchmark datasets and cutting-edge architectures demonstrate the efficacy and generality of TAFAS, especially in long-term forecasting scenarios that suffer from significant distribution shifts. The code is available at https://github.com/kimanki/TAFAS.
Source link
lol
Battling the Non-stationarity in Time Series Forecasting via Test-time Adaptation
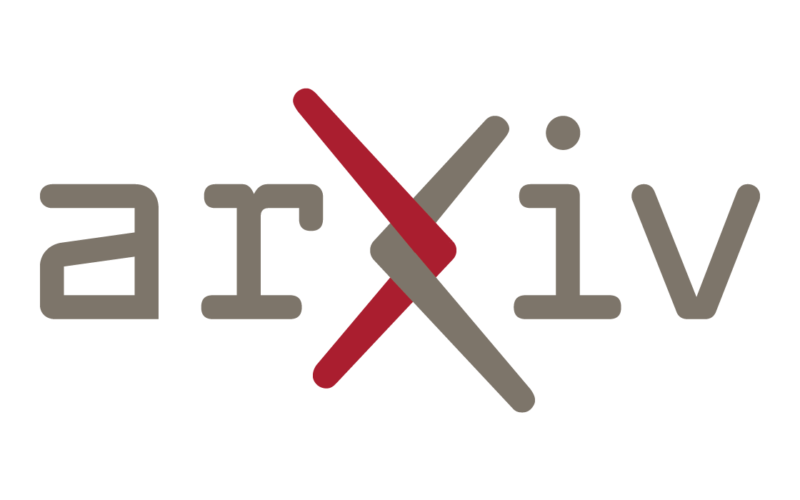