arXiv:2501.04952v1 Announce Type: new
Abstract: As AI systems become more capable, widely deployed, and increasingly autonomous in critical areas such as cybersecurity, biological research, and healthcare, ensuring their safety and alignment with human values is paramount. Machine unlearning — the ability to selectively forget or suppress specific types of knowledge — has shown promise for privacy and data removal tasks, which has been the primary focus of existing research. More recently, its potential application to AI safety has gained attention. In this paper, we identify key limitations that prevent unlearning from serving as a comprehensive solution for AI safety, particularly in managing dual-use knowledge in sensitive domains like cybersecurity and chemical, biological, radiological, and nuclear (CBRN) safety. In these contexts, information can be both beneficial and harmful, and models may combine seemingly harmless information for harmful purposes — unlearning this information could strongly affect beneficial uses. We provide an overview of inherent constraints and open problems, including the broader side effects of unlearning dangerous knowledge, as well as previously unexplored tensions between unlearning and existing safety mechanisms. Finally, we investigate challenges related to evaluation, robustness, and the preservation of safety features during unlearning. By mapping these limitations and open challenges, we aim to guide future research toward realistic applications of unlearning within a broader AI safety framework, acknowledging its limitations and highlighting areas where alternative approaches may be required.
Source link
lol
Open Problems in Machine Unlearning for AI Safety
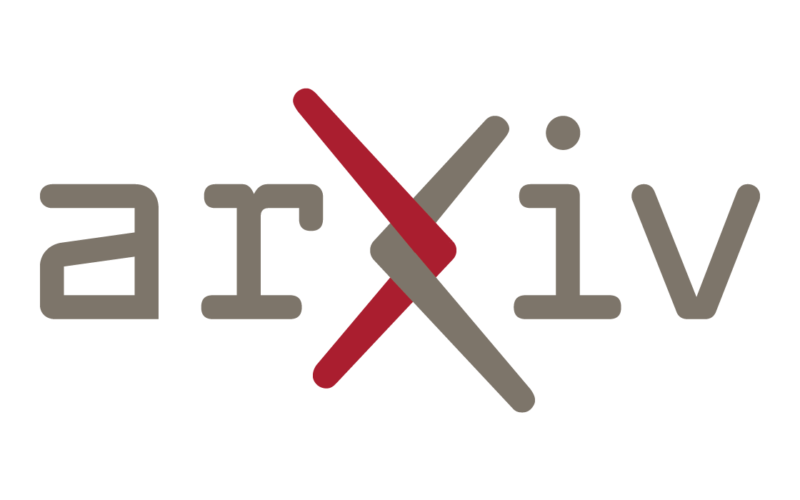