arXiv:2501.00303v1 Announce Type: new
Abstract: The primary challenge of cross-domain few-shot segmentation (CD-FSS) is the domain disparity between the training and inference phases, which can exist in either the input data or the target classes. Previous models struggle to learn feature representations that generalize to various unknown domains from limited training domain samples. In contrast, the large-scale visual model SAM, pre-trained on tens of millions of images from various domains and classes, possesses excellent generalizability. In this work, we propose a SAM-aware graph prompt reasoning network (GPRN) that fully leverages SAM to guide CD-FSS feature representation learning and improve prediction accuracy. Specifically, we propose a SAM-aware prompt initialization module (SPI) to transform the masks generated by SAM into visual prompts enriched with high-level semantic information. Since SAM tends to divide an object into many sub-regions, this may lead to visual prompts representing the same semantic object having inconsistent or fragmented features. We further propose a graph prompt reasoning (GPR) module that constructs a graph among visual prompts to reason about their interrelationships and enable each visual prompt to aggregate information from similar prompts, thus achieving global semantic consistency. Subsequently, each visual prompt embeds its semantic information into the corresponding mask region to assist in feature representation learning. To refine the segmentation mask during testing, we also design a non-parameter adaptive point selection module (APS) to select representative point prompts from query predictions and feed them back to SAM to refine inaccurate segmentation results. Experiments on four standard CD-FSS datasets demonstrate that our method establishes new state-of-the-art results. Code: https://github.com/CVL-hub/GPRN.
Source link
lol
SAM-Aware Graph Prompt Reasoning Network for Cross-Domain Few-Shot Segmentation
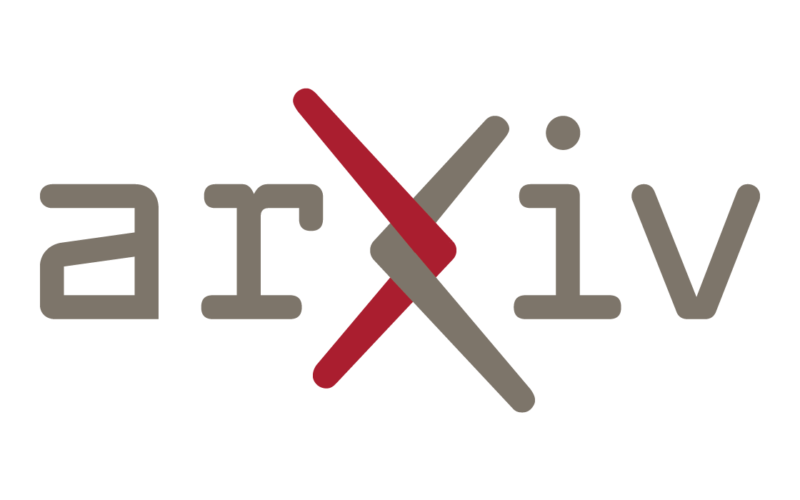