arXiv:2412.12153v1 Announce Type: new
Abstract: Model merging aims to build a multi-task learner by combining the parameters of individually fine-tuned models without additional training. While a straightforward approach is to average model parameters across tasks, this often results in suboptimal performance due to interference among parameters across tasks. In this paper, we present intriguing results that weight averaging implicitly induces task vectors centered around the weight averaging itself and that applying a low-rank approximation to these centered task vectors significantly improves merging performance. Our analysis shows that centering the task vectors effectively separates core task-specific knowledge and nuisance noise within the fine-tuned parameters into the top and lower singular vectors, respectively, allowing us to reduce inter-task interference through its low-rank approximation. We evaluate our method on eight image classification tasks, demonstrating that it outperforms prior methods by a significant margin, narrowing the performance gap with traditional multi-task learning to within 1-3%
Source link
lol
Revisiting Weight Averaging for Model Merging
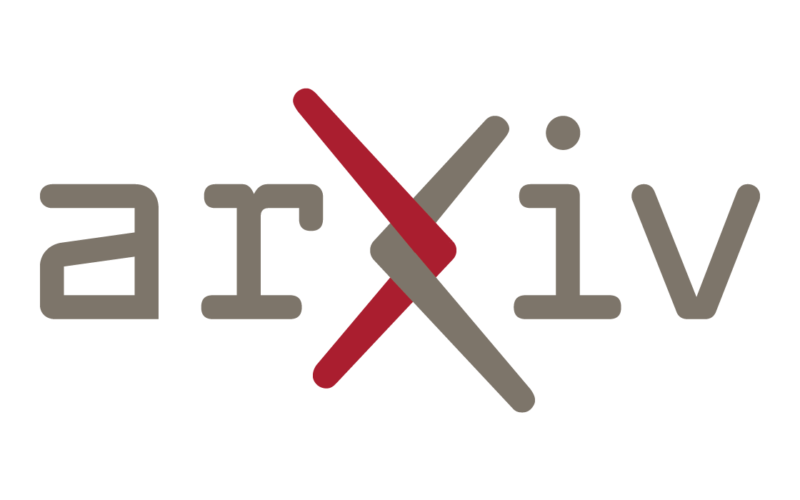