arXiv:2412.12147v1 Announce Type: new
Abstract: Generalizing across robot embodiments and tasks is crucial for adaptive robotic systems. Modular policy learning approaches adapt to new embodiments but are limited to specific tasks, while few-shot imitation learning (IL) approaches often focus on a single embodiment. In this paper, we introduce a few-shot behavior cloning framework to simultaneously generalize to unseen embodiments and tasks using a few (emph{e.g.,} five) reward-free demonstrations. Our framework leverages a joint-level input-output representation to unify the state and action spaces of heterogeneous embodiments and employs a novel structure-motion state encoder that is parameterized to capture both shared knowledge across all embodiments and embodiment-specific knowledge. A matching-based policy network then predicts actions from a few demonstrations, producing an adaptive policy that is robust to over-fitting. Evaluated in the DeepMind Control suite, our framework termed modelname{} demonstrates superior few-shot generalization to unseen embodiments and tasks over modular policy learning and few-shot IL approaches. Codes are available at href{https://github.com/SeongwoongCho/meta-controller}{https://github.com/SeongwoongCho/meta-controller}.
Source link
lol
Meta-Controller: Few-Shot Imitation of Unseen Embodiments and Tasks in Continuous Control
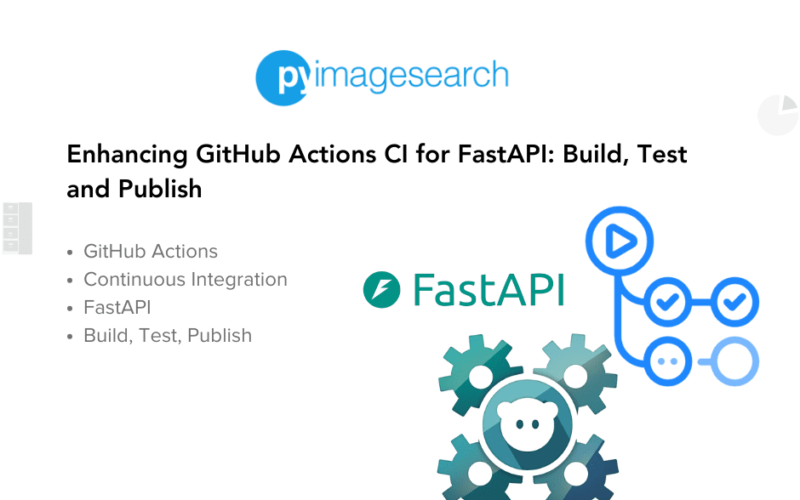