arXiv:2412.07435v1 Announce Type: cross
Abstract: In recent years, there has been a surge of interest in proving discretization bounds for sampling under isoperimetry and for diffusion models. As data size grows, reducing the iteration cost becomes an important goal. Inspired by the great success of the parallel simulation of the initial value problem in scientific computation, we propose parallel Picard methods for sampling tasks. Rigorous theoretical analysis reveals that our algorithm achieves better dependence on dimension $d$ than prior works in iteration complexity (i.e., reduced from $widetilde{O}(log^2 d)$ to $widetilde{O}(log d)$), which is even optimal for sampling under isoperimetry with specific iteration complexity. Our work highlights the potential advantages of simulation methods in scientific computation for dynamics-based sampling and diffusion models.
Source link
lol
Parallel simulation for sampling under isoperimetry and score-based diffusion models
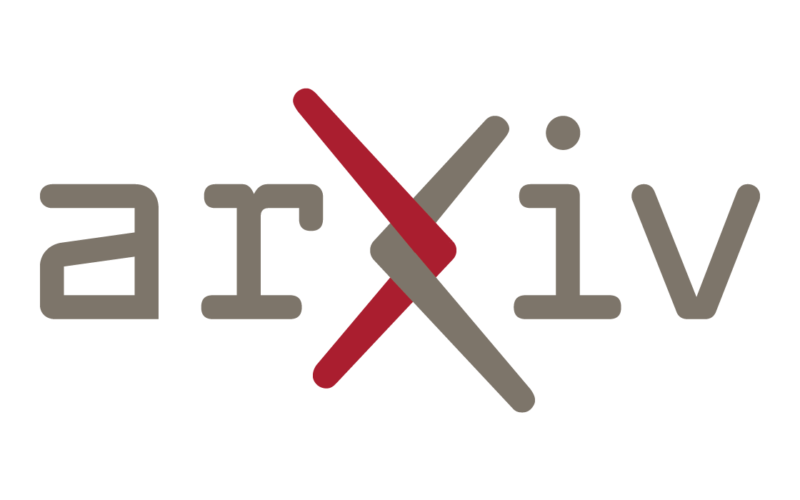