arXiv:2411.07180v1 Announce Type: cross
Abstract: Understanding and manipulating the causal generation mechanisms in language models is essential for controlling their behavior. Previous work has primarily relied on techniques such as representation surgery — e.g., model ablations or manipulation of linear subspaces tied to specific concepts — to intervene on these models. To understand the impact of interventions precisely, it is useful to examine counterfactuals — e.g., how a given sentence would have appeared had it been generated by the model following a specific intervention. We highlight that counterfactual reasoning is conceptually distinct from interventions, as articulated in Pearl’s causal hierarchy. Based on this observation, we propose a framework for generating true string counterfactuals by reformulating language models as Generalized Structural-equation. Models using the Gumbel-max trick. This allows us to model the joint distribution over original strings and their counterfactuals resulting from the same instantiation of the sampling noise. We develop an algorithm based on hindsight Gumbel sampling that allows us to infer the latent noise variables and generate counterfactuals of observed strings. Our experiments demonstrate that the approach produces meaningful counterfactuals while at the same time showing that commonly used intervention techniques have considerable undesired side effects.
Source link
lol
Counterfactual Generation from Language Models
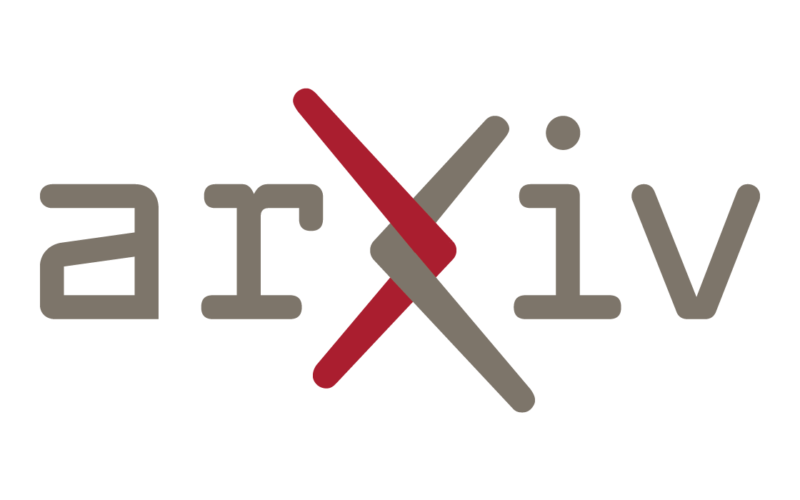