arXiv:2411.13590v1 Announce Type: new
Abstract: Surprisingly a number of Earth’s waterways remain unmapped, with a significant number in low and middle income countries. Here we build a computer vision model (WaterNet) to learn the location of waterways in the United States, based on high resolution satellite imagery and digital elevation models, and then deploy this in novel environments in the African continent. Our outputs provide detail of waterways structures hereto unmapped. When assessed against community needs requests for rural bridge building related to access to schools, health care facilities and agricultural markets, we find these newly generated waterways capture on average 93% (country range: 88-96%) of these requests whereas Open Street Map, and the state of the art data from TDX-Hydro, capture only 36% (5-72%) and 62% (37%-85%), respectively. Because these new machine learning enabled maps are built on public and operational data acquisition this approach offers promise for capturing humanitarian needs and planning for social development in places where cartographic efforts have so far failed to deliver. The improved performance in identifying community needs missed by existing data suggests significant value for rural infrastructure development and better targeting of development interventions.
Source link
lol
Deep learning waterways for rural infrastructure development
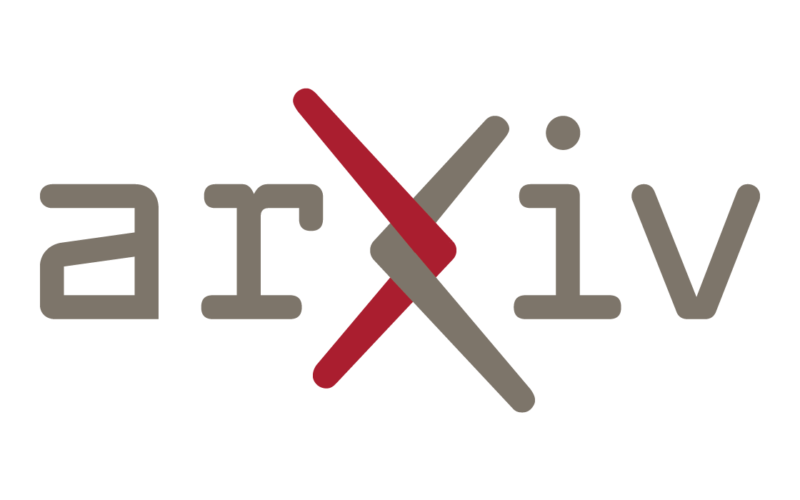