arXiv:2409.15371v1 Announce Type: new
Abstract: As Large Language Models (LLMs) continue to grow in size, their computational and memory requirements increase correspondingly. Consequently, the exploration of cost-effective and efficient fine-tuning methods has become increasingly important. Low-Rank Adaptation (LoRA) has achieved remarkable training results by freezing the original weights and training only low-rank matrices, establishing itself as the predominant fine-tuning method for LLMs. In pursuit of performance closer to full-parameter training, a series of LoRA variants have emerged, such as LoRA+, PISSA, Olora, and LoRA-GA. However, these methods also make the fine-tuning initialization process more complex, and it remains challenging to surpass the performance ceiling of full fine-tuning. To address these issues, this paper introduces an innovative method called Bone (Block Affine), which not only reduces memory overhead but also emphasizes the internal connections between weights, leading to faster convergence and better data fitting. Experimental comparisons across two different LLM architectures (LLaMA2, RWKV6) and various parameter scales demonstrate that the Bone structure can achieve rapid convergence and superior data fitting without the need for complex initialization. For example, when fine-tuning LLaMA2-7B on the MetaMathQA dataset and validating on GSM8k and math benchmarks, Bone achieved fine-tuning scores of 49.36 and 8.8, respectively, outperforming PISSA by 5.84% and 1.96%.
Source link
lol
Bone: Block Affine Transformation as Parameter Efficient Fine-tuning Methods for Large Language Models
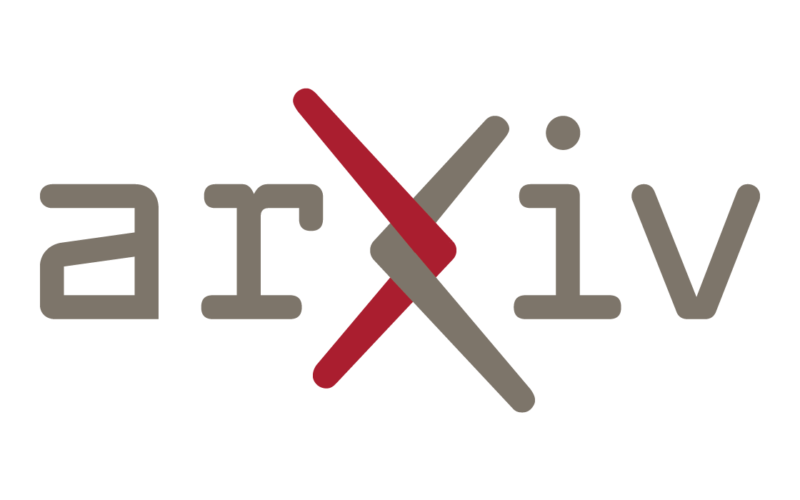