arXiv:2412.00073v1 Announce Type: new
Abstract: The rise of advanced AI models like Generative Adversarial Networks (GANs) and diffusion models such as Stable Diffusion has made the creation of highly realistic images accessible, posing risks of misuse in misinformation and manipulation. This study evaluates the effectiveness of convolutional neural networks (CNNs), as well as DenseNet architectures, for detecting AI-generated images. Using variations of the CIFAKE dataset, including images generated by different versions of Stable Diffusion, we analyze the impact of updates and modifications such as Gaussian blurring, prompt text changes, and Low-Rank Adaptation (LoRA) on detection accuracy. The findings highlight vulnerabilities in current detection methods and propose strategies to enhance the robustness and reliability of AI-image detection systems.
Source link
lol
Addressing Vulnerabilities in AI-Image Detection: Challenges and Proposed Solutions
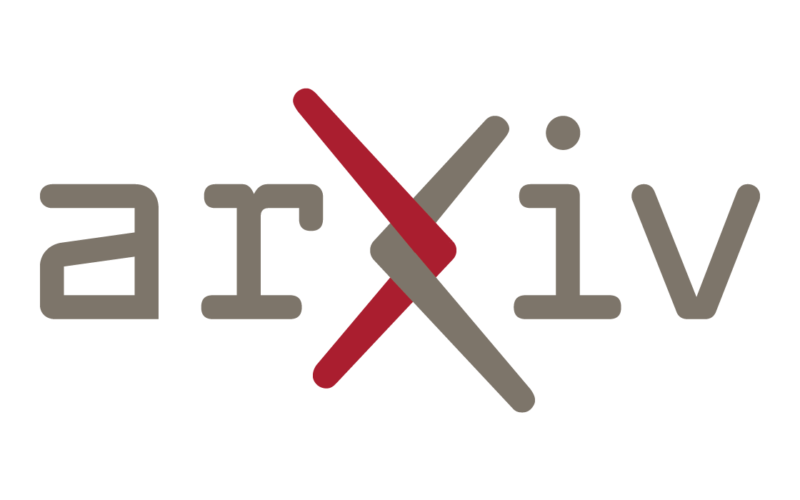