(AI Generated/Shutterstock)
A recent Goldman Sachs report said the lack of a “killer app” for generative AI beyond chatbots and co-pilots could hinder its adoption. What GenAI needs, the analysts wrote, were AI-infused applications that could take actions by themselves. Could a new model type, dubbed the large action model, or LAM, fit the bill?
The LAM concept started to emerge in late 2023 as a natural follow-on to large language models (LLMs), which have caught the eyes of the world for the human-like text responses they can generate. LAMs go beyond the text generation capabilities of an LLM by actually executing some action within a software program.
“LLMs are good at one way interchange of ‘Here’s my question, answer me,’” says Pankaj Chawla, chief innovation officer at Virginia-based tech consultancy 3Pillar. “But what do I do with it after that? That’s where the magic of large action models come into play.”
3Pillar is building LAMs for clients that see the value in LLMs, but want to take the next step and automate repetitive tasks to achieve a higher return on their investment, says Chawla, who goes by PC.
LAMs execute actions using existing programmatic pathways, such as APIs, or in some cases interacting directly with the user interface of an application, which is similar to robotic process automation (RPA), he says.
For instance, if an executive is taking a business trip, a LAM could be built to respond to the human instruction “Find me economy-plus flights and a four-star hotel for Milan, Italy, from October 10 through the 17th.” The LAM could not only respond to that request with suggestions, but also navigate the necessary systems and call the necessary data to secure reservations.
Another way to think about LAMS is they pick up where co-pilots leave off, PC says.
“A co-pilot is in my in my view something you’re still interacting with as a human, but you’re not stitching together multiple things to do together to carry out an outcome, a business outcome or a personal outcome,” he tells Datanami. “Co-pilot goes a little bit in that direction, but [LAM] is about creating a self-learning script, and as it does that action more than once, it gets better at it.”
Not all companies use the same terminology. Gartner, for example, calls it neurosymbolic AI, which is the combination of neural nets and symbolic programming (i.e. traditional deterministic programming).
Amazon and its AWS subsidiary have invested substantially in developing what they call semi-autonomous agents, which go beyond coding co-pilots to handle basic coding tasks. Andy Jassy, the former AWS head who took over for Jeff Bezos two years ago, recently said these agents have saved the company 4,500 developer-years in upkeep of its Java code.
Another LAM example is the Rabbit r1, which is a GPT-3.5-based personal assistant that implements a LAM style interface to enable automated interactions with certain sites, including Spotify, Apple Music, Midjourney, Suno, Uber, and DoorDash.
Apple Intelligence, currently in preview, is another example of a LAM-type system, as is what Salesforce is doing with its enterprise computing suite, PC says. “Salesforce has been talking about using LAMs to work behind the scenes with their Salesforce data to carry out a series of actions, like launching a campaign and actually tracking the outputs,” he says.
In July, McKinsey published a report titled “Why agents are the next frontier of generative AI” that extolled the potential of agents to power the next generation of GenAI.
“We are beginning an evolution from knowledge-based, gen-AI-powered tools–say, chatbots that answer questions and generate content–to gen AI–enabled ‘agents’ that use foundation models to execute complex, multistep workflows across a digital world,” analysts with the consulting giant write. “In short, the technology is moving from thought to action.”
AI agents, McKinsey says, will be able to automate “complex and open-ended use cases” thanks to three characteristics they possess, including: the capability to manage multiplicity; the capability to be directed by natural language; and the capability to work with existing software tools and platforms.
These “hyper-efficient virtual coworkers,” as McKinsey calls them, could soon be seen in the wild in specific arenas, like loan underwriting, code documentation and modernization, and online marketing campaign creation.
“Although agent technology is quite nascent, increasing investments in these tools could result in agentic systems achieving notable milestones and being deployed at scale over the next few years,” the company writes.
PC acknowledges that there are some challenges to building automated applications with the LAM architecture at this point. LLMs are probabilistic and sometimes can go off the rails, so it’s important to keep them on track by combining them with classical programming using deterministic techniques.
For example, 3Pillar is currently developing a LAM application that interacts with people and asks them questions, but the LLM sometimes drifts off or suggests things that aren’t legal.
“So it’s the deterministic programming that keeps it on track, keeps it [within] the guardrails, but it still leverages the LLMs power,” he says. “We run knowledge graphs behind the scenes so …the answers are much more focused, precise and not hallucinated because it’s going against that data set.”
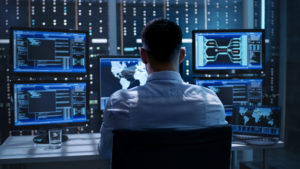
Reptititive tasks done by human employees can potentially be automated by a combination of probabilistic and deterministic programming (Gorodenkoff/Shutterstock)
Backoffice applications might be the best testing ground for LAMs, as they don’t expose the company to as much liability from an LLM going off the rails, PC says. Integrated ERP suites from large software companies have access to lots of cross-industry data and cross-discipline workflows, which will inform and drive LAMs and agent-based AI.
The LAM is just an architectural concept today, but over time, the concept will be fleshed out and there will be software-based frameworks that companies can use to accelerate the development of LAM and AI agent systems, PC says.
“I think there’ll be more frameworks that let you get there with predefined integrations, calls, whatever for commonly used systems, very much like adapters are for enterprise service buses like you see today,” he says. “So there may be an adapter for Oracle for this and that and APIs that are available to carry out actions, and then frameworks to actually build and create those actions through more through configuration and point and click versus code.”
However, the potential upside with consumer-based LAMs and autonomous AI agents is truly massive, and it’s just a matter of time before consumers start seeing these in the wild, PC says.
“I see this on a horizon for the next two to five years,” he says. “You will start to see these kind of applications that are real, AI-driven solutions coming in [where] the chatbot and LLM are just building blocks. We still have issues with hallucinations and everything like that. But I foresee two to five years before we start to see real world applications.”
Related Items:
Is the GenAI Bubble Finally Popping?
Source link
lol