arXiv:2408.14595v1 Announce Type: new
Abstract: Multimodal foundation models (MFMs) such as OFASys show the potential to unlock analysis of complex data such as images, videos, and audio data via text prompts alone. However, their performance may suffer in the face of text input that differs even slightly from their training distribution, which is surprising considering the use of modality-specific data to “ground” the text input. This study demonstrates that prompt instability is a major concern for MFMs, leading to a consistent drop in performance across all modalities, but that instability can be mitigated with additional training with augmented data. We evaluate several methods for grounded prompt perturbation, where we generate perturbations and filter based on similarity to text and/or modality data. After re-training the models on the augmented data, we find improved accuracy and more stable performance on the perturbed test data regardless of perturbation condition, suggesting that the data augmentation strategy helps the models handle domain shifts more effectively. In error analysis, we find consistent patterns of performance improvement across domains, suggesting that retraining on prompt perturbations tends to help general reasoning capabilities in MFMs.
Source link
lol
Surprisingly Fragile: Assessing and Addressing Prompt Instability in Multimodal Foundation Models
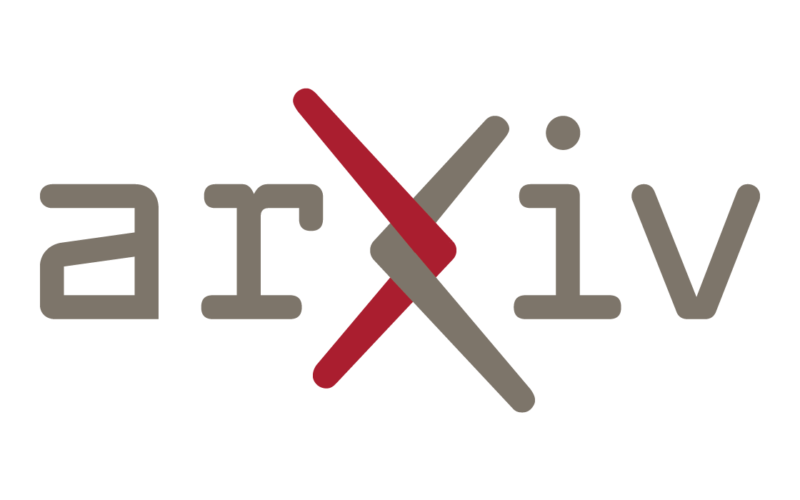