arXiv:2408.07889v1 Announce Type: new
Abstract: Existing RGB-T tracking algorithms have made remarkable progress by leveraging the global interaction capability and extensive pre-trained models of the Transformer architecture. Nonetheless, these methods mainly adopt imagepair appearance matching and face challenges of the intrinsic high quadratic complexity of the attention mechanism, resulting in constrained exploitation of temporal information. Inspired by the recently emerged State Space Model Mamba, renowned for its impressive long sequence modeling capabilities and linear computational complexity, this work innovatively proposes a pure Mamba-based framework (MambaVT) to fully exploit spatio-temporal contextual modeling for robust visible-thermal tracking. Specifically, we devise the long-range cross-frame integration component to globally adapt to target appearance variations, and introduce short-term historical trajectory prompts to predict the subsequent target states based on local temporal location clues. Extensive experiments show the significant potential of vision Mamba for RGB-T tracking, with MambaVT achieving state-of-the-art performance on four mainstream benchmarks while requiring lower computational costs. We aim for this work to serve as a simple yet strong baseline, stimulating future research in this field. The code and pre-trained models will be made available.
Source link
lol
MambaVT: Spatio-Temporal Contextual Modeling for robust RGB-T Tracking
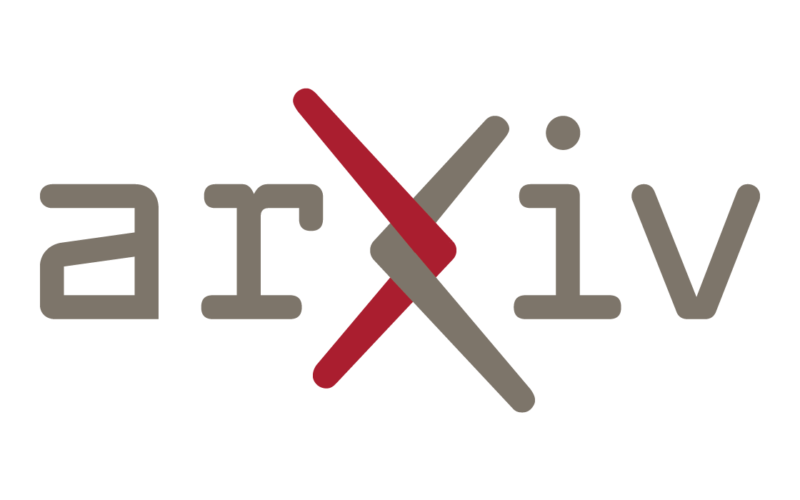