arXiv:2407.18402v1 Announce Type: new
Abstract: In this paper, we develop an unsupervised learning approach to earthquake detection. We train a specific class of deep auto-encoders that learn to reproduce the input waveforms after a data-compressive bottleneck, and then use a simple triggering algorithm at the bottleneck to label waveforms as noise or signal.
Our approach is motivated by the intuition that efficient compression of data should represent signals differently from noise, and is facilitated by a time-axis-preserving approach to auto-encoding and intuitively-motivated choices on the architecture and triggering.
We demonstrate that the detection performance of the unsupervised approach is comparable to, and in some cases better than, some of the state-of-the-art supervised methods. Moreover, it has strong emph{cross-dataset generalization}. By experimenting with various modifications, we demonstrate that the detection performance is insensitive to various technical choices made in the algorithm.
Our approach has the potential to be useful for other signal detection problems with time series data.
Source link
lol
The seismic purifier: An unsupervised approach to seismic signal detection via representation learning
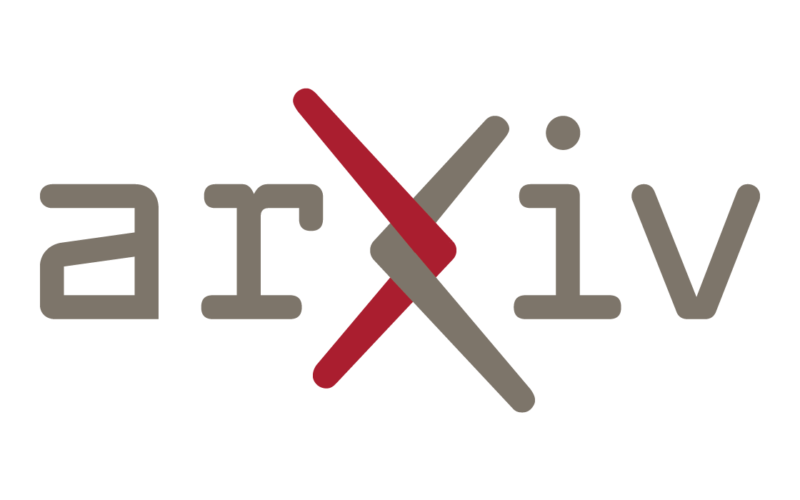