arXiv:2407.07279v1 Announce Type: new
Abstract: State space models (SSMs) have shown remarkable empirical performance on many long sequence modeling tasks, but a theoretical understanding of these models is still lacking. In this work, we study the learning dynamics of linear SSMs to understand how covariance structure in data, latent state size, and initialization affect the evolution of parameters throughout learning with gradient descent. We show that focusing on the learning dynamics in the frequency domain affords analytical solutions under mild assumptions, and we establish a link between one-dimensional SSMs and the dynamics of deep linear feed-forward networks. Finally, we analyze how latent state over-parameterization affects convergence time and describe future work in extending our results to the study of deep SSMs with nonlinear connections. This work is a step toward a theory of learning dynamics in deep state space models.
Source link
lol
Towards a theory of learning dynamics in deep state space models
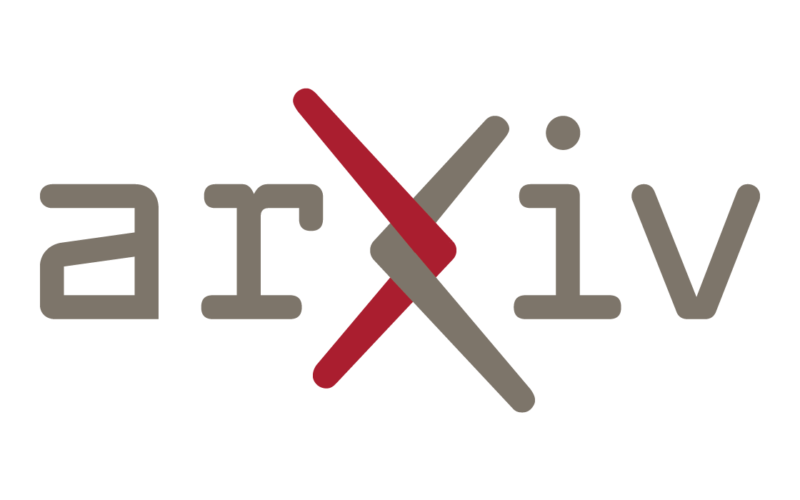