arXiv:2407.04969v1 Announce Type: new
Abstract: Summarization is a fundamental task in natural language processing (NLP) and since large language models (LLMs), such as GPT-4 and Claude, come out, increasing attention has been paid to long-form summarization whose input sequences are much longer, indicating more information contained.
The current evaluation metrics either use similarity-based metrics like ROUGE and BERTScore which rely on similarity and fail to consider informativeness or LLM-based metrics, lacking quantitative analysis of information richness and are rather subjective.
In this paper, we propose a new evaluation metric called EVA-Score using Atomic Fact Chain Generation and Document-level Relation Extraction together to automatically calculate the informativeness and give a definite number as an information score. Experiment results show that our metric shows a state-of-the-art correlation with humans. We also re-evaluate the performance of LLMs on long-form summarization comprehensively from the information aspect, forecasting future ways to use LLMs for long-form summarization.
Source link
lol
EVA-Score: Evaluation of Long-form Summarization on Informativeness through Extraction and Validation
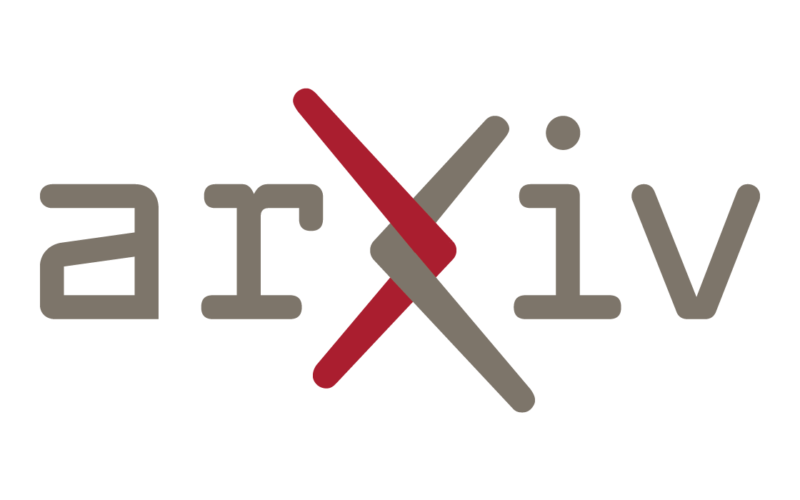