arXiv:2407.03436v1 Announce Type: new
Abstract: The environments where individuals live can present diverse navigation challenges, resulting in varying navigation abilities and strategies. Inspired by differing urban layouts and the Dual Solutions Paradigm test used for human navigators, we developed a simulated navigation environment to train deep reinforcement learning agents in a shortcut usage task. We modulated the frequency of exposure to a shortcut and navigation cue, leading to the development of artificial agents with differing abilities. We examined the encoded representations in artificial neural networks driving these agents, revealing intricate dynamics in representation learning, and correlated them with shortcut use preferences. Furthermore, we demonstrated methods to analyze representations across a population of nodes, which proved effective in finding patterns in what would otherwise be noisy single-node data. These techniques may also have broader applications in studying neural activity. From our observations in representation learning dynamics, we propose insights for human navigation learning, emphasizing the importance of navigation challenges in developing strong landmark knowledge over repeated exposures to landmarks alone.
Source link
lol
A Role of Environmental Complexity on Representation Learning in Deep Reinforcement Learning Agents
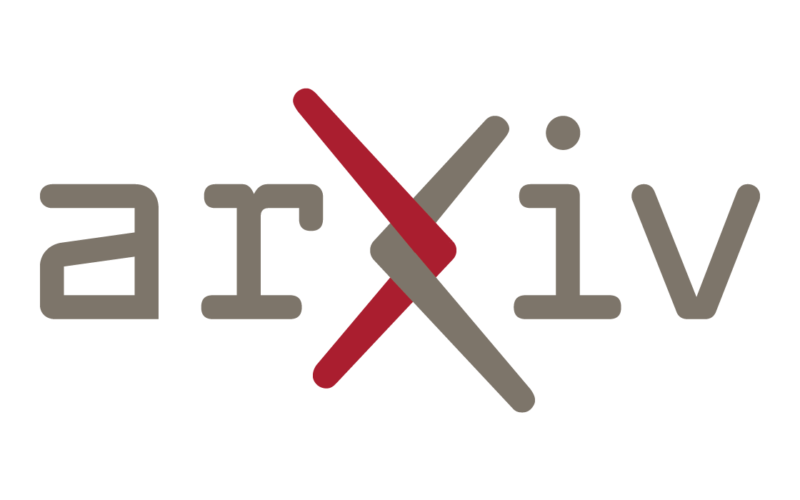