Snowflake Inc.’s Data Cloud Summit 2024 was a weeklong event that brought together more than 20,000 of Snowflake’s core enterprise customers, developers and ecosystem partners to San Francisco this past week.
The event highlighted the expanding aspirations of the company as it evolves its Data Cloud into an AI Data Cloud and a platform for developing intelligent data apps. Snowflake Data Cloud Summit 2024 marks the next chapter in the company’s history with new Chief Executive Sridhar Ramaswamy taking center stage, driving even faster product innovation and an ambitious total available market expansion strategy. Snowflake has moved beyond being a friendly and simpler data warehouse to tackling new problems, including how to bring AI value to enterprise customers, helping ecosystem partners monetize, and providing tools and services to build artificial intelligence-powered enterprise data apps.
In this Breaking Analysis, we summarize the top takeaways from the summit. We’ll share our thoughts on the announcements, innovations, competitive implications, challenges and opportunities Snowflake faces. And we’ll share our expectations for Databricks Inc.’s Data + AI Summit next week. Finally, we’ll show you a snapshot the data platform ecosystem with some spending profiles of key players.
Snowflake evolves from cloud data warehouse to AI-powered platform for building data apps
What is Snowflake? Is it a data cloud? An AI company? Or is Snowflake a data application platform? The answer is it is all of those things. We’re no longer talking about just databases and data warehouses. Rather, we’re viewing Snowflake as a platform to build and run intelligent applications.
Snowflake started out as a platform for building analytic artifacts such as business intelligence dashboards. Databricks, by contrast, started out catering to the data science audience. However, all applications are increasingly driven by analytics, and the way you steer the analytics is with data. So, in our view, we’re seeing an infusion of analytics throughout more of what were operational applications. We see this as a big transition for Snowflake.
Changing competitive landscape: Databricks, Microsoft, Salesforce
The implication is the competitive landscape is changing. Snowflake’s expanding aspirations are bringing it into new markets with new competitors, beyond cloud data warehouses. The company is wading into the territory of firms such as Microsoft Corp. and Salesforce Inc.
Databricks key points: Access data via any compute engine – through its catalog
- Snowflake’s vision: To be the “iPhone of data apps,” enabling a new breed of applications. Its main focus is simplicity and building a comprehensive and easy-to-use platform.
- Databricks’ vision: Focuses on the separation of compute from data, supporting diverse data engines, including data processing, streaming, SQL analytics, machine learning training and serving, foundation model training and serving, and catalog-based governance.
Snowflake aims to revolutionize the data application landscape by becoming the “iPhone of data apps.” This vision is different in our view from Databricks’ strategy.
First, Databricks is focusing on being the best platform for building analytic artifacts that stand alone or get embedded in larger applications. Second, Databricks doesn’t have one integrated, powerful compute engine to address as many workloads as Snowflake. Instead, it separated its specialized processing engines, starting with Spark, from the open Delta Lake data storage.
As a result, Databricks was the first to integrate governance functionality, in Unity Catalog, directly with its data lakehouse. That enables other tools and engines to work with data in the data lake without going through a Databricks database management system. In other words, Databricks is offering specialization and choice, requiring somewhat greater developer and administrative sophistication for most workloads.
Because Snowflake always aspired to serve applications, not just analytics, it built a database that could support more workloads. This year’s Summit showed this strategy bearing fruit as functionality promised in the engine over the last couple of years finally showed up in functionality for developers, data scientists, AI engineers, data engineers, data analysts and even business users. And it’s all integrated in an iPhone-like App Store-like marketplace where enterprises and ecosystem partners can publish their apps and data assets.
Microsoft key points: Lead with the simplicity of its corporate developer tools
- Microsoft’s low-code toolchain:
- Power BI, Power Apps, Power Automate, Power Pages, and Copilot Studio.
- Unified toolchain for corporate developers across the entire product line.
- Snowflake’s tools:
- Various developer experiences including…
- Streamlit, Cortex AI Studio, Cortex Analyst, Cortex Search, ML Studio, Notebooks, Copilot.
- Less unified low-code development toolchain.
- Platform comparison:
- Snowflake has a unified data platform that supports all data types and most workloads
- Microsoft leads with the appeal of its tools for corporate developers. Microsoft’s Fabric offers a “good enough” data platform with a variety of compute engines similar to Databricks.
Though Snowflake’s data engine achieves its power and simplicity through integration, it can’t match Microsoft with that same type of differentiation with developer tooling. Microsoft provides a comprehensive low-code/no-code toolchain through Power BI, Power Apps, Power Automate, Power Pages and Copilot Studio, integrated not just across Fabric but all of Azure, Dynamics and Office.
Salesforce as a new competitor
We believe that Salesforce, a pioneer in software as a service, has significantly evolved its platform, now serving 150,000 customers. It has become a dominant player in customer management, from the largest enterprises to small businesses, covering a broad spectrum of needs such as outbound and inbound sales, call centers and field service. Salesforce has developed a data platform with low-code tools and an integrated customer 360 data model, shifting much of the traditional data engineering work into a configuration-driven, point-and-click environment.
Key points: Leverage its installed base and build in a Customer 360 model
- Salesforce’s market position:
- Pioneered SaaS.
- Serves 150,000 customers.
- Dominates customer management
- Applications and services:
- Outbound sales.
- Inbound call centers.
- Field service.
- Data platform evolution:
- Developed a comprehensive data platform with low-code tools.
- Integrated in a customer 360 data model.
- Shifts traditional data engineering to configuration-driven, no-code/low-code solutions.
- Integration and smart analytics:
- Automatically updates analytic models based on customer interactions.
- Enhanced analytics through integration with operational applications.
Salesforce has significantly evolved its platform and can leverage its installed base of 150,000 customers to become a major presence with its data platform. It has become the dominant customer relationship management vendor, from the largest enterprises to small businesses, covering outbound and inbound sales, call centers and field service. It has developed a data platform built on an integrated customer 360 data model, shifting much of the traditional data engineering work into a configuration-driven, point-and-click environment. And it has the low code/no code tools to complement the platform.
Salesforce has transitioned from a pioneer in SaaS to a comprehensive platform that integrates customer management and analytics. By incorporating low-code tools and a customer a 360 data model, Salesforce simplifies data engineering tasks, enabling smarter analytics through integration with operational applications. This evolution underscores Salesforce’s adaptability and potential to fence out Snowflake from its customer 360 data corpus.
Challenge: Maintain iPhone simplicity with open data formats
Snowflake has responded to the customer call for open data formats. Customers tell theCUBE Research that they want to control their data, they don’t want lock-in and they’re navigating to open formats such as Iceberg. The idea is they want to be able to use any compute engine and bring it to their data. The challenge for Snowflake (and customers) is how to gain the benefits of integration while at the same time ensuring governance, privacy and security across data estates.
Snowflake’s brand promise is “Move the data into Snowflake and it will be integrated, governed and safe.” This is very much iPhone-like in the experience. However, open formats introduce a number of challenges, including:
- Which governance technology to use. Snowflake announced it is open-sourcing Polaris, a technical metadata catalog — while at the same time keeping Horizon, its robust governance framework, which manages its proprietary Native Tables.
- If customers choose to use Managed Iceberg Tables (Snowflake’s integrated Iceberg capability) they can achieve partial openness. External tools and engines can read these tables without going through the Snowflake DBMS.
- At the same time, if customers choose to stay outside of the Snowflake framework and choose other governance tools, they’ll still be able to read the external data from Snowflake.
Snowflake’s strategy in this regard is to offer Polaris as an open-source tool, and attract customers via its superb data engine.
How will bundled pricing affect Snowflake’s goal of accommodating ‘all data, all workloads?’
Our research indicates that a critical challenge for Snowflake is rationalizing its margin model while competing with software-only data engineering offerings. Last year’s Snowflake Summit revealed that some customers are performing data pipeline, data science and data engineering work outside Snowflake to minimize compute engine costs. Despite Snowflake’s integrated model supporting streaming, advanced analytics, data engineering and generative AI, cost concerns remain prevalent.
Snowflake’s contention is that doing the data engineering and data science work inside Snowflake remains a superior cost option. But because Snowflake bundles cloud costs into its pricing, it may appear to be more expensive.
TheCUBE Research to data has not done an extensive economic analysis of this issue, but it remains on our agenda to do so.
Key points
- Customer behavior:
- Some customers perform data tasks outside Snowflake to reduce compute costs.
- Snowflake’s integrated model:
- Supports streaming, advanced analytics, data engineering and generative AI in a single, integrated platform.
- Cost comparison:
- Databricks does not include cloud costs from its pricing, unlike Snowflake.
- Internal analysis by Snowflake suggests competitive costs, but detailed public analysis is lacking.
- Market positioning:
- Snowflake in our view must maintain a roughly 77% gross margin.
- Pricing needs to balance between gross margin and market share retention.
- Competitor strategies:
- Databricks and Starburst offer software-only solutions.
- Customers using these solutions still incur hardware costs through Amazon Web Services or Microsoft Azure and must consider these in a comprehensive manner to truly understand the benefits.
A key financial challenge for Snowflake is balancing its pricing strategy to achieve sufficient gross margins while staying competitive in the data engineering market. The integrated model’s benefits must outweigh the compute costs to retain customers who might otherwise opt for software-only solutions and manage hardware costs separately. The need for transparent analysis of Snowflake’s pricing compared to competitors is essential to substantiate its value proposition.
Great plumbing (architecture) matters
Our belief is that Snowflake is now surfacing the power of many years of underlying architectural development work and building value on top of its system. This is evident through advancements such as data catalogs, Snowflake Polaris (an open-source project) and Horizon (a heavy governance tool). Additionally, gen AI additions fit well into the model, as will future enhancements.
Several key announcements from Snowflake over the past 12 to 24 months are now generally available or nearing GA status. These developments include Snowpark Container Services, which enables the integration of any compute into the Snowflake ecosystem. As well, the company has made significant enhancements such as the open data Iceberg tables with the Polaris catalog, Cortex Gen AI and a native notebook experience for data scientists.
On balance, these put Snowflake in a strong competitive position, building on the strength of its data engine.
Digging into Snowflake’s architecture
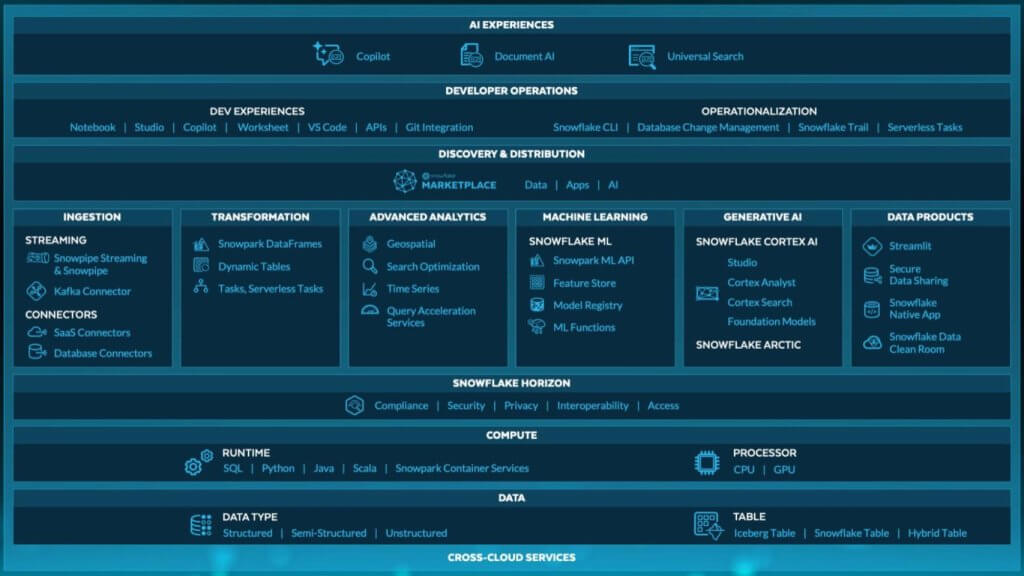
The eye chart above depicts Snowflake’s architectural components. We’ll take a moment to talk about how it all works together.
You’ve got the multicloud layer at the bottom. Snowflake was one of the first to, to develop the multicloud strategy. We called it supercloud with a single global namespace even though most people are running in individual clouds. Nonetheless, it works well on multiple clouds. And it’s pretty much the same experience. Snowflake supports multiple data types. It has streaming integrated and one place to develop, deploy and operate.
In the next layer above, we see ingest, transform and Snowflake can incrementally update. The platform includes sophisticated analytics, time series capabilities and much more.
Then it goes to machine learning, it takes raw data and turns it into metrics. For example, what type of engagements are occurring, what’s the click through and so forth. All of this updates automatically compressed timeframes and granularity. Now it’s adding gen AI, which was a big focus at Summit this year, with Cortex and fine-tuning models.
Snowflake is all in on bringing Nvidia Corp. capabilities to its platforms. It has NeMo (neural modules) for retrieval and improving RAG. Now It’s building in NIMS (Nvidia Inference Microservices) and they make this all presentable and governed.
And on top of that, Snowflake is building a marketplace with partners and a marketplace for data. And they’ve got the developer and operational tooling above that, which is integrated. Then of course, AI on top of that to make things easier for end users.
Taken together, we believe this demonstrates how Snowflake leverages the power of simplicity and integration in many use cases. For example, let’s take an e-commerce personalization scenario. By streaming real-time data from e-commerce applications and enabling low-latency reactions, Snowflake provides a unified platform for developing, deploying and operating personalized user experiences. This addresses challenges associated with multiple product ecosystems, which complicate development and increase latency.
Key capabilities of the Snowflake platform
- Real-time personalization:
- Streaming data (e.g. e-commerce applications) for real-time personalization.
- Unified platform for developing, deploying and operating personalized experiences.
- Low-latency data ingestion and processing:
- Snowpipe streaming for low latency data ingestion.
- Dynamic tables that function as materialized views, chaining transformations efficiently.
- Comprehensive data utilization:
- Integration of session data with historical and contextual data.
- Adjustable latency for data refresh, approaching real-time updates.
- AI and machine learning integration:
- Feeding real-time data into machine learning models for product recommendations.
- Use of gen AI for tasks like text summarization, sentiment analysis, translation and text generation.
- Nvidia partnership enhances AI capabilities with advanced inference microservices (NIMS).
- Emerging semantic layer:
- Future opportunities include the development of a semantic layer for natural language querying.
- Integration of semantic layers into the engine for richer data querying and analysis.
- Future enhancements to define metrics and dimensions, learning from natural language queries.
On balance, Snowflake’s comprehensive and integrated approach to data streaming, low-latency processing and AI integration significantly enhances the ability to personalize user experiences in real time. The development of a semantic layer for natural language querying would in our view, further Snowflake’s position in the market and provide leverage with advanced technologies, optimizing its data platform for seamless user experiences and operational efficiency.
Finding NeMo and NIMS
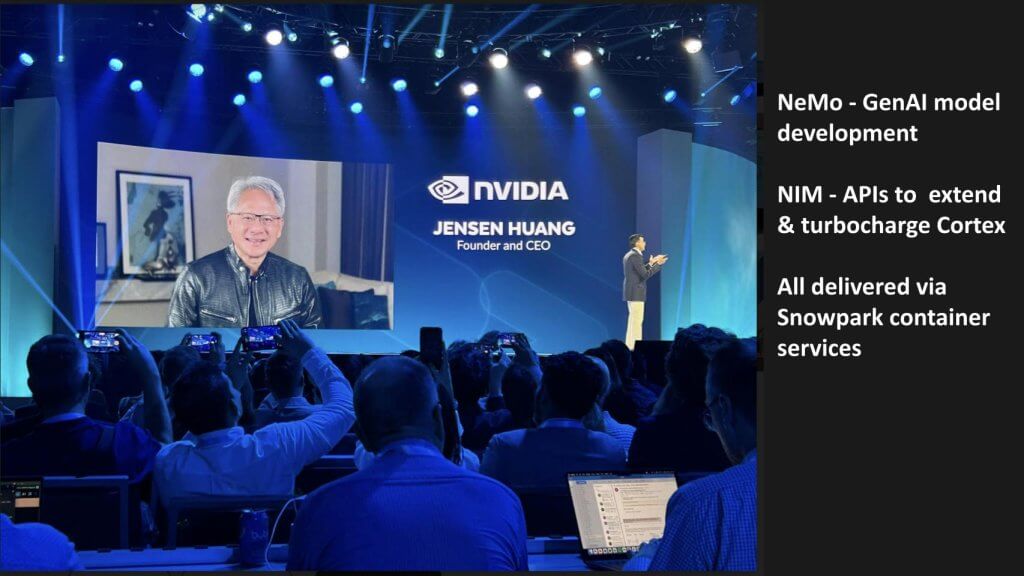
Snowflake continues to intensify its collaboration with Nvidia. CEO Jensen Huang was showcased at Snowflake Summit but was remote from Taiwan after his big reveal of a new chip at Computex. The partnership with Nvidia is based on the integration of Nvidia’s advanced computing capabilities into Snowflake’s platform, particularly through the introduction of NeMo for gen AI model development and NIMS for application programming interface enhancements. These advancements leverage Snowpark Container Services, enhancing Snowflake’s developer platform with powerful tools for model training and deployment.
Key points
- Nvidia collaboration:
- Jensen Huang’s remote participation follows up from last year’s in-person conversation with Frank Slootman.
- Introduction of NeMo for containerized Gen AI model development.
- NIMS APIs enhance and turbocharge Cortex.
- Snowpark container services:
- Facilitates the integration of advanced AI tools from Nvidia.
- Provides a rich developer platform leveraging Snowflake’s compute engine.
- Model training and fine-tuning:
- Nvidia tools allow pretraining and fine-tuning models within Cortex.
- Simplified, serverless fine-tuning for models like Llama and Mistral.
- Integration of specialized Nvidia models accessible via SQL or Python functions.
- Industry innovations – Snowflake is not alone:
- Advancements such as Red Hat’s InstructLab simplify model fine-tuning.
- IBM’s research contributes to advanced, self-training models.
- Intense competition benefits customers with a diverse array of solutions.
The collaboration between Snowflake and Nvidia brings cutting-edge AI capabilities to Snowflake’s data platform, significantly enhancing its developer tools and simplifying model training and deployment. This partnership, enabled by Snowpark Container Services, underscores the dynamic and innovative nature of the data platform industry, ultimately benefiting customers with more advanced and efficient solutions.
Tip of the Iceberg
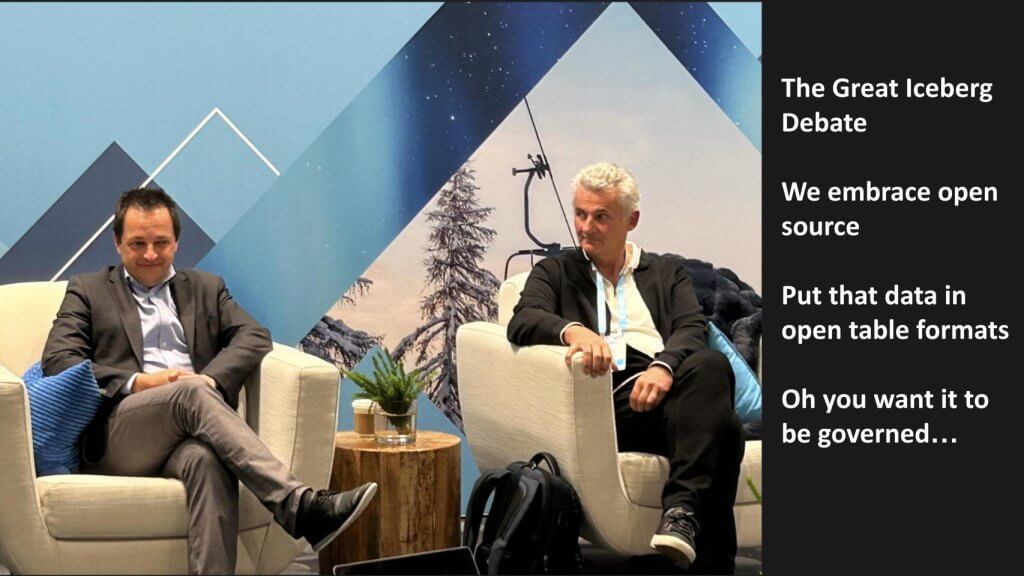
Throughout the week at Snowflake Summit we’ve been covering what we coined “The Great Iceberg Debate.” Our friend Sanjeev Mohan helped frame the issue.
Our collective analysis highlights the intense debate around Iceberg open table formats. This issue was intensified by Databricks’ announcement of acquiring Tabular just as Snowflake’s visionary founder Benoit Dageville was walking onstage for his keynote on Tuesday. The acquisition emphasizes Databricks’ commitment to leading the open table formats issue, a mantle also embraced by Snowflake.
Snowflake’s goal is to meet customer demands for open data formats and expand its technical metadata catalog, Polaris by open sourcing the project. Snowflake’s strategy is to offer an open-source technical metadata solution, while at the same time offering Managed Iceberg services as first-class citizens with enhanced governance capabilities via its Horizon offering.
Key points
- Debate on open table formats:
- Databricks announced the acquisition of Tabular to strengthen its position in open table formats and took a shot across the bow at Snowflake.
- Snowflake’s commitment to open table formats is evident.
- Snowflake’s strategy:
- Open-sourcing Polaris, the technical metadata catalog.
- Offer managed Iceberg tables within Snowflake for enhanced governance.
- Flexibility to read from external tables without updating them (unmanaged).
- Industry context:
- Historical shifts in the definition of “open,” with comparisons to Unix systems.
- Market trends towards open data formats allowing any compute to access any data.
- Comparison with Databricks:
- Databricks’ Unity Catalog approach versus Snowflake’s open-source Polaris catalog. Databrick’s is trying to bring a fully governed experience in an effort to enable any compute engine to access any governed data.
- Challenges faced by Databricks in achieving interoperability among Delta, Iceberg and Hudi tables, leads to the Tabular acquisition, further confusing the situation.
- Snowflake’s competitive edge:
- Promote Polaris to provide a base level of standardization and compute on the basis of its engine.
- Strongest compute engine capabilities.
- Strategic focus on leveraging core strengths to maintain market leadership.
- Databricks’ strategic edge:
- Less mature compute engine.
- An attempt to enable any compute engine to access fully governed table formats.
The debate over open table formats underscores the evolving strategies of key players like Snowflake and Databricks. Snowflake’s approach of offering both open-source and managed solutions through Polaris and Iceberg managed solutions highlights its commitment to flexibility and customer choice. Meanwhile, Databricks’ acquisition of Tabular signals its efforts to overcome interoperability challenges and strengthen its open data format capabilities. This competitive landscape, driven by advancements in open data formats, on the one hand could benefit customers seeking versatile and efficient data solutions. On the other hand, it could further confuse and fork the market.
A spending snapshot of the data ecosystem
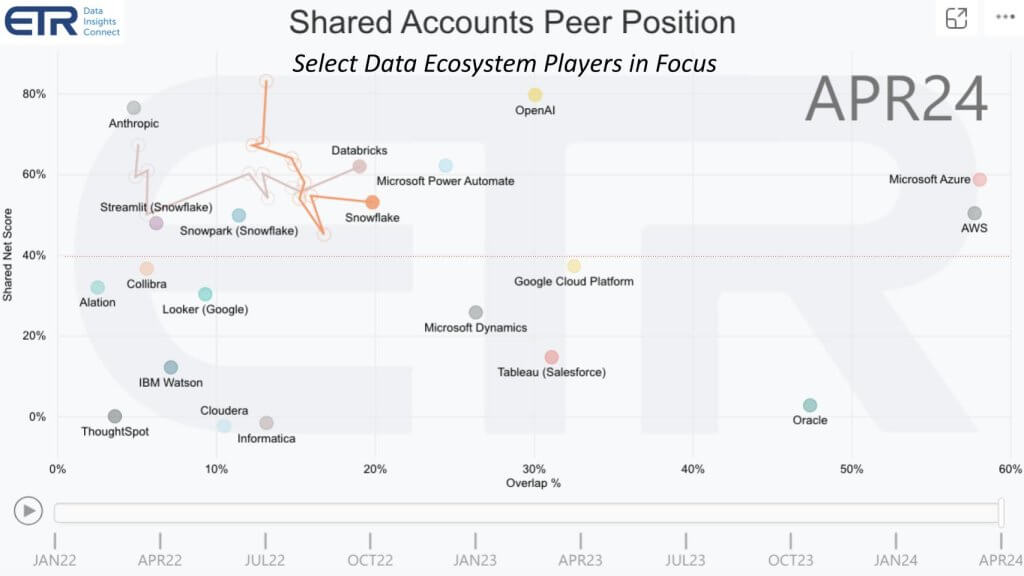
In the above Enterprise Technology Research slide, we’ve selected a representative sample of data ecosystem players. This is by no means comprehensive, but we wanted to give you a sense of what’s happening for various sectors and put them in context.
On the vertical axis we show Net Score, which is a measure of spending momentum. And in the horizontal axis is the presence or Overlap within about 1,800 information technology decision maker respondents in the April survey. Anything over that 40% line on the vertical axis is considered highly elevated. You can see we’ve plotted the big three cloud vendors – Azure and AWS obviously up and to the right. We’ve added in some other products that ETR has in its taxonomy, such as Microsoft Dynamics, and we put in Power Automate, even though that’s a robotic process automation tool, but we wanted to represent the power line.
We show several business intelligence vendors, the visualization with Tableau, owned by Salesforce. We have ThoughtSpot Inc. and Google LLC’s Looker also representing the BI tool vendors. We’ve added some governance with Alation Inc. and Collibra Inc. And, and of course we’ve got OpenAI and Anthropic PBC because the large language model vendors will play a role here. And we include Oracle Corp. for context as well as IBM Corp.’s watson and Cloudera Inc. And there are dozens more companies we could have included in this ecosystem, which is rich and growing.
We highlight Databricks and Snowflake and you can see the trajectory of those two companies over time with the squiggly lines. We’ve talked about the convergence of these two companies from a spending profile standpoint in past episodes of Breaking Analysis.
We’re seeing data become the center of the infrastructure debate. Everyone needs to get access to the data. Many need to write the data, not just read it. And that’s why we’re moving to this world where data is an open resource and we’re up-leveling where the competition is to the engines that read and write that data. So that’s why we put forth the vision of the sixth data platform where we have to separate compute from data and allow any compute to access any data.
What to watch for at Databricks Data + AI Summit
TheCUBE will be back at Moscone next week for Databricks’ big customer event. Here are some of the things we’ll be watching:
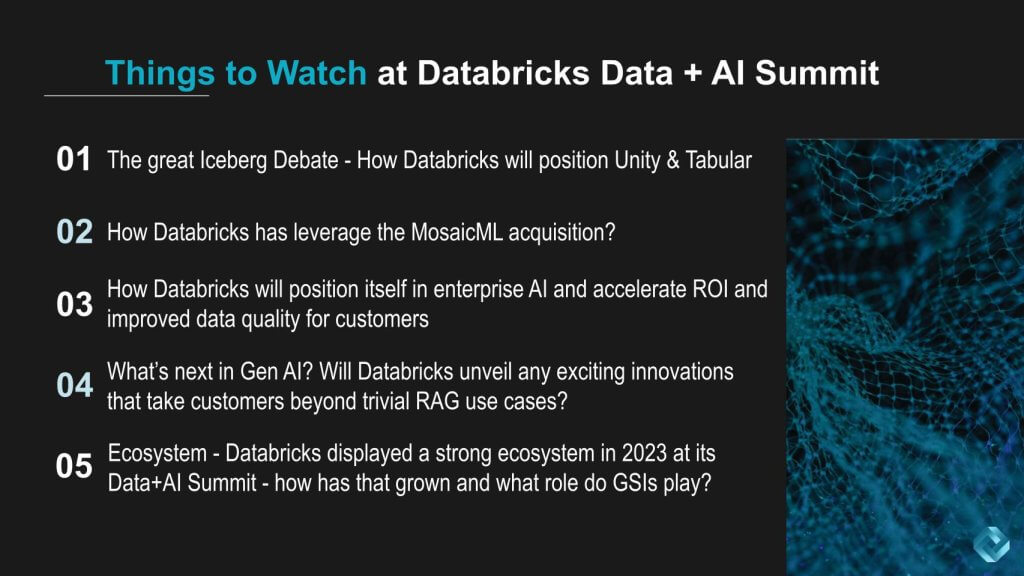
Our analysts will be watching the ongoing developments in the competitive landscape between Snowflake and Databricks, particularly concerning the recent acquisition of Tabular by Databricks. We expect this move, alongside past strategic acquisitions such as MosaicML, will be highlighted. We also expect Databricks to demonstrate how it’s getting leverage from past acquisitions and bundling innovation from these firms into its offerings.
Key expectations
- Acquisitions and strategic moves:
- Databricks’ acquisition of Tabular and MosaicML.
- What innovations have they built on top of MosaicML and how will they position Tabular?
- Enterprise AI and data quality:
- Databricks’ positioning in enterprise AI to enhance ROI, value and data quality.
- The importance of trust and reliability in AI deployments.
- Generative AI innovations:
- Anticipation of new innovations in generative AI from Databricks.
- Moving beyond retrieval-augmented generation of RAG use cases to more advanced capabilities.
- Potential integration of multiple AI models.
- Ecosystem and partnerships:
- The role of global system integrators in the Databricks ecosystems.
- The strong ecosystems observed at last year’s event.
- Company aspirations:
- Snowflake’s ambition to be a data-centric cloud platform.
- Databricks’ focus on building analytic artifacts that integrate with platforms like Salesforce and Azure, rather than becoming a full application platform.
- Databricks as a horizontal capability across any compute engine.
The competitive dynamics between Snowflake and Databricks are intensifying and will continue, driven by strategic acquisitions and evolving aspirations in the AI and data landscape. While Snowflake aims to become a comprehensive AI data cloud, Databricks focuses on providing versatile analytic artifacts for integration with other platforms. These strategies, alongside strong ecosystems and partnerships, underscore the rapid innovation and strategic maneuvers shaping the future of enterprise AI and data solutions.
Stop by and see theCUBE team and bring your perspectives to the audience – we’d love to see you!
Keep in touch
Your vote of support is important to us and it helps us keep the content FREE.
One click below supports our mission to provide free, deep, and relevant content.
Join our community on YouTube
Join the community that includes more than 15,000 #CubeAlumni experts, including Amazon.com CEO Andy Jassy, Dell Technologies founder and CEO Michael Dell, Intel CEO Pat Gelsinger, and many more luminaries and experts.
THANK YOU
Source link
lol