The competition in the data industry is intensifying as Databricks Inc. vies for dominance. The company has thrown down the gauntlet by open-sourcing its Unity Catalog.
Data giants Databricks and Snowflake Inc. hosted events within a week of each other. Last week’s Data + AI Summit showcased Databricks’ increasing momentum and emphasis on collaboration. More than 66% of the contributions to Spark are coming from outside Databricks, according to Rob Strechay (pictured, left), principal analyst at theCUBE Research. The company’s announcement that it was open sourcing Unity, a unified governance solution for data and artificial intelligence, follows news of Snowflake’s upcoming release of Polaris, an open-source Iceberg catalog. These developments suggest that the competition grounds have moved from data formats to data catalogs.
TheCUBE analysts Rob Strechay, George Gilbert and John Furrier discuss insights from the Data + AI Summit.
“Databricks is saying, ‘Forget the data format now. We got that covered. You won’t have to care. The new platform is the catalog,’” George Gilbert (middle), principal analyst for data and AI at theCUBE, said during an analysis segment at the Data + AI Summit. “Microsoft is kind of weak there, and the Snowflake catalog is tied to their compute engine. So they’re trying to get everyone to plug in, because once you plug in all your tools, the functionality of your tools depends on what’s in the catalog.”
Strechay and Gilbert were joined by John Furrier (right), theCUBE Research executive analyst, in one of several analyst segments at the Data + AI Summit 2024. They discussed how Databricks is going on the offensive with a strategy based on data interoperability and integrating generative AI throughout its platform.
Here’s the complete video of theCUBE’s Data + AI Summit “AnalystANGLE”:
1. Unity Catalog could give Databricks a competitive advantage against Snowflake.
Unlike Snowflake, which ensures all data stays inside its platform, Databricks has never owned the data and is now pursuing an open format strategy that could put Snowflake on the back foot and move the point of control from the database to the catalog.
“Databricks went from being five years behind in DBMS technology to being many years ahead in catalog technology,” said Gilbert in a keynote analysis of the summit’s first day. “Where Snowflake open-sourced the Polaris Catalog for a governance tool for Iceberg tables, it’s just Iceberg and it’s just technical metadata — whereas Unity is all your data assets even beyond Delta. Basically, they changed the playing field [from] something that favored Snowflake to something that favored Databricks.”
Databricks recently acquired Tabular Technologies Inc. to accelerate interoperability between Delta Lake and Iceberg, two leading data formats. Gilbert compares this move to the first level in Maslow’s hierarchy of needs, with rationalizing the two formats as food and shelter, then moving up to Unity, which manages data quality and lineage and, eventually, semantic harmonization between the different types of data as self-actualization.
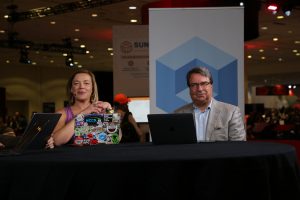
TheCUBE analysts Savannah Peterson and John Furrier talk about how Databricks is transforming data governance.
“This is the evolution of Databricks. Their track record from day one with Spark was constantly popping on that next lily pad and getting to the next innovation,” said Furrier, who has been following the company since early days. “You’re seeing massive tooling. You’re seeing the Delta Lake uniform, Unity Catalog got governance … Ali Ghodsi really wants to push the democratization. How he’s doing that is by forcing the standard of table interoperability, data formats.”
As the complete technical metadata operational catalog, Unity builds on Databricks’ relationship with its user base. By creating interoperability across different data formats and sources, the company is hoping to better serve its customers’ data engineering teams.
“They own data science; they own those guys. That’s their key persona that they’ve been huge with with AI in general,” said Strechay in a day 2 keynote analysis. “Now they’re looking at it with … Delta Lake and what they’ve been doing there and SQL being kind of first-class citizen, the progress they’ve made with it and now bringing in some of the things like LakeFlow Connect.”
Along with Tabular, Databricks recently acquired Mosaic AI and might be aiming to be the go-to AI operating system, Furrier theorized. But even with Databricks’ new acquisitions, there is a long way to go before AI can perform the multilayered reasoning companies are looking for.
“Gen AI may be attracting all, but to get it right is a very, very tough job. We are now finally facing it, after almost two years of trying to do it,” said Sanjeev Mohan, principal at SanjMo, in an analyst segment with Tony Baer, principal at dbInsight LLC. “The idea was, in 2023, we’ll do experimentation. In 2024, we’ve got production. But they’re nowhere close to being production. NLMs are amazing for basic language task[s], like summarization, recommendations. But when it comes to doing reasoning and more complex tasks, which is what the customers want, at the end of the day, we still have a lot of work to do.”
Here are theCUBE’s complete keynote analyses:
2. Databricks’ industry partnerships are expanding as the company homes in on AI.
Databricks’ acquisition of Mosaic AI reflects the company’s ongoing efforts to be a generative AI platform for a range of enterprises. Its goal is to help customers bring together all of their data regardless of format, according to Joel Minnick, vice president of marketing at Databricks.
“[Mosaic AI]’s that end-to-end workflow from the time that I get the data prepped to how I build the model, to how I deploy the model, to how I then ultimately evaluate whether or not the model’s successful,” he said in an interview with theCUBE. “The role of Unity Catalog is to underlie that.”
Small language models have been gaining popularity, with businesses wanting to prioritize efficiency and interoperability. Compound systems of AI models are also being favored with customers because of their speed, according to Minnick.
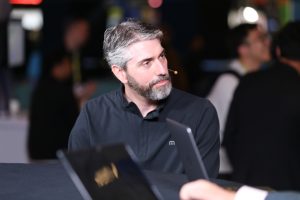
Databricks’ Joel Minnick discusses the company’s acquisition of Mosaic AI.
“We are finding for the customers who are starting to put things into production, particularly on newer use cases, like putting AI agents out there into the world now or multimodal systems out there, the compound systems [are] moving much faster,” he said. “[There’s] a dense model, which means I have one single model tackling the entire problem from the time that I got the prompt from the user to the time I give you the answer back. Compound AI says, ‘Well, let’s actually break it up into discrete units.’”
Databricks continues to enhance its network of partnerships in order to prepare customers for the AI era, including collaborations with Fivetran, a data movement platform, and Alation, which helps customers catalog and organize their data assets.
“You need to have your data foundation really, really ready to move into the gen AI world. That’s easy to say, really, really hard to do,” said Jeff Veis, chief marketing officer of Impetus Technologies, which assists enterprises in moving data off legacy platforms into Databricks. “You need to be able to have it be governed; it needs to be able to be consumed and done in a trusted way. Only 30% of Databricks customers are on catalog today.”
Data observability and modernized data infrastructure are crucial for companies trying to implement AI models, which require good data to produce reliable answers. Data silos are an ongoing problem, as well as accessibility. To address these issues, Databricks has partnered with Prophecy Inc. to create an AI co-pilot that gives its users the tools they need to harness their data.
“Everybody who wants data has got to have access to it … we are providing the tooling layer that makes data engineers … and data analysts and [a] line of business users, all of them productive with data,” said Raj Bains, founder and chief executive officer of Prophecy, in an interview. “As Databricks is anchored on and focused on data functionality, we are anchored and focused on the data user.”
Here’s the complete interview with Joel Minnick:
3. Databricks is applying AI to diverse use cases.
A number of Databricks’ partners were present at the Data + AI Summit, and they showcased increasingly varied use cases for the data engineering and AI tools that Databricks offers. With their data in order, companies such as Condé Nast, which hosts over 60 publications, can now build language models that detect user preferences and service them accordingly.

Condé Nast’s Tim Shokair talks about the company’s AI use cases.
“Our data engineering team is processing all our data and storing it within Databricks, making it accessible for us,” said Tim Shokair, senior director of data science at Condé Nast, in an interview with theCUBE. “My team uses it for model development. We do a lot of model exploration. We use a lot of the ML tools that they built in, and then my machine learning engineering team uses it now to take those models, turn them into services. What I get really excited about is thinking about how well these models have to understand content to make these predictions, and that’s generally what I like to exploit.”
Although the platform has a steeper learning curve than Snowflake, Databricks is popular among data scientists because it has unlimited scalability for large amounts of data and provides users a workspace with its machine learning runtime, Managed MLflow.
“It started for me as a data scientist, a tool that we could easily use to collaborate and building off of Spark, being able to process massive amounts of data or build models on top of massive amounts of data was really useful,” Shokair said. “Then, they’ve just continued to build features that make it easier for us as data scientists and machine learning engineers to put models into production.”
One use case for Condé Nast was creating a tool for the Vogue Runway app that allows users to search 1.2 million Runway images.
Having a strategy for integrating AI into your business is just as crucial as having the funds or the technology at your disposal, according to Anand Pradhan, senior director of technology at the AI Center of Excellence at ICE, which works with companies including Mosaic AI and Databricks to support companies’ AI journeys.
“We make sure that we implement the AI use cases properly, so that each [step] of the process is followed so that we get some value addition first of all, and it is implemented the right way … that’s one thing,” Pradhan said, who also runs the New York Stock Exchange Launchpad Lab. “The other thing is having a strategic vision in the company to lay out the foundation so that multiple AI applications or projects can run with the same vision.”
Here’s theCUBE’s complete interview with Tim Shokair:
To watch more of SiliconANGLE and theCUBE Research’s coverage of Data + AI Summit, here’s our complete video playlist:
https://www.youtube.com/watch?v=videoseries
Photo: SiliconANGLE
Your vote of support is important to us and it helps us keep the content FREE.
One click below supports our mission to provide free, deep, and relevant content.
Join our community on YouTube
Join the community that includes more than 15,000 #CubeAlumni experts, including Amazon.com CEO Andy Jassy, Dell Technologies founder and CEO Michael Dell, Intel CEO Pat Gelsinger, and many more luminaries and experts.
THANK YOU
Source link
lol