arXiv:2407.00148v1 Announce Type: new
Abstract: Anomaly detection and localization in medical imaging remain critical challenges in healthcare. This paper introduces Spatial-MSMA (Multiscale Score Matching Analysis), a novel unsupervised method for anomaly localization in volumetric brain MRIs. Building upon the MSMA framework, our approach incorporates spatial information and conditional likelihoods to enhance anomaly detection capabilities. We employ a flexible normalizing flow model conditioned on patch positions and global image features to estimate patch-wise anomaly scores. The method is evaluated on a dataset of 1,650 T1- and T2-weighted brain MRIs from typically developing children, with simulated lesions added to the test set. Spatial-MSMA significantly outperforms existing methods, including reconstruction-based, generative-based, and interpretation-based approaches, in lesion detection and segmentation tasks. Our model achieves superior performance in both distance-based metrics (99th percentile Hausdorff Distance: $7.05 pm 0.61$, Mean Surface Distance: $2.10 pm 0.43$) and component-wise metrics (True Positive Rate: $0.83 pm 0.01$, Positive Predictive Value: $0.96 pm 0.01$). These results demonstrate Spatial-MSMA’s potential for accurate and interpretable anomaly localization in medical imaging, with implications for improved diagnosis and treatment planning in clinical settings. Our code is available at~url{https://github.com/ahsanMah/sade/}.
Source link
lol
Localizing Anomalies via Multiscale Score Matching Analysis
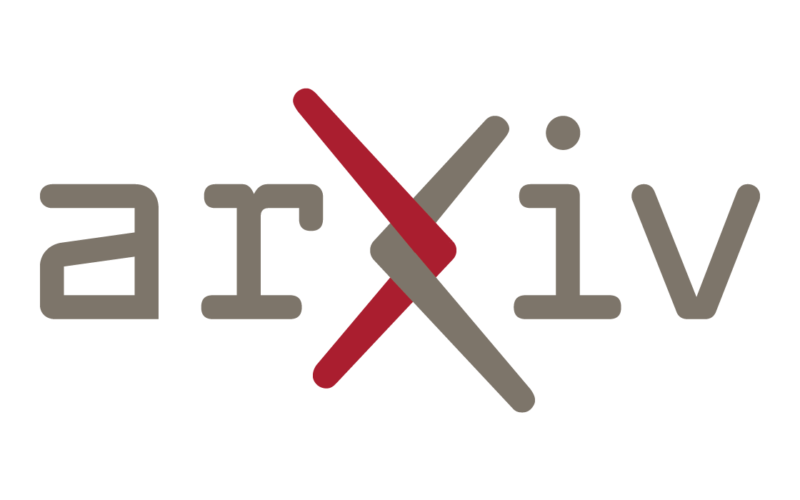