arXiv:2406.18800v1 Announce Type: new
Abstract: Common infinite-width architectures such as Neural Tangent Kernels (NTKs) have historically shown weak performance compared to finite models. This has been attributed to the absence of feature learning. We show that this is not the case. In fact, we show that infinite width NTK models are able to access richer features than finite models by selecting relevant subfeatures from their (infinite) feature vector. In fact, we show experimentally that NTKs under-perform traditional finite models even when feature learning is artificially disabled. Instead, weak performance is due to the fact that existing constructions depend on weak optimizers like SGD. We provide an infinite width limit based on ADAM-like learning dynamics and demonstrate empirically that the resulting models erase this performance gap.
Source link
lol
Infinite Width Models That Work: Why Feature Learning Doesn’t Matter as Much as You Think
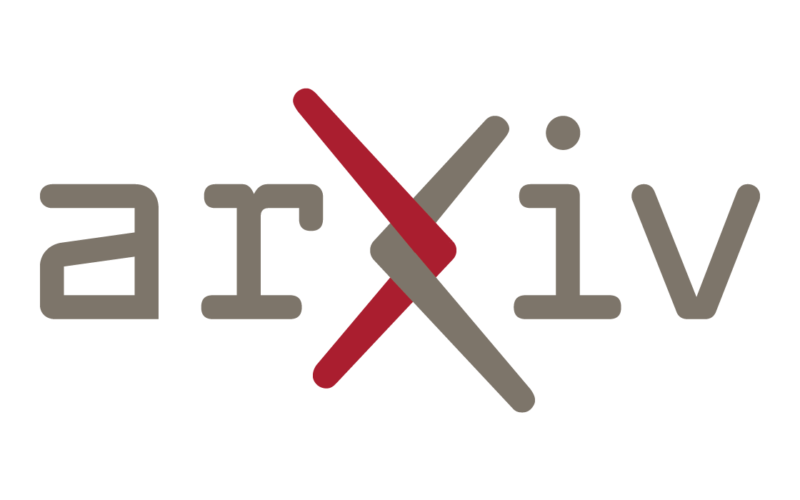