arXiv:2406.18541v1 Announce Type: new
Abstract: In recent years, point cloud normal estimation, as a classical and foundational algorithm, has garnered extensive attention in the field of 3D geometric processing. Despite the remarkable performance achieved by current Neural Network-based methods, their robustness is still influenced by the quality of training data and the models’ performance. In this study, we designed a fundamental framework for normal estimation, enhancing existing model through the incorporation of global information and various constraint mechanisms. Additionally, we employed a confidence-based strategy to select the reasonable samples for fair and robust network training. The introduced sample confidence can be integrated into the loss function to balance the influence of different samples on model training. Finally, we utilized existing orientation methods to correct estimated non-oriented normals, achieving state-of-the-art performance in both oriented and non-oriented tasks. Extensive experimental results demonstrate that our method works well on the widely used benchmarks.
Source link
lol
Refining 3D Point Cloud Normal Estimation via Sample Selection
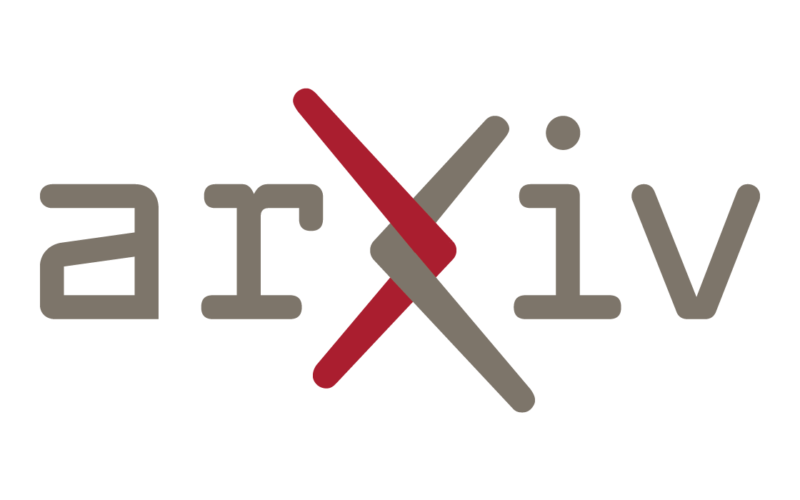