arXiv:2406.17890v1 Announce Type: new
Abstract: We propose a novel approach that enhances multivariate function approximation using learnable path signatures and Kolmogorov-Arnold networks (KANs). We enhance the learning capabilities of these networks by weighting the values obtained by KANs using learnable path signatures, which capture important geometric features of paths. This combination allows for a more comprehensive and flexible representation of sequential and temporal data. We demonstrate through studies that our SigKANs with learnable path signatures perform better than conventional methods across a range of function approximation challenges. By leveraging path signatures in neural networks, this method offers intriguing opportunities to enhance performance in time series analysis and time series forecasting, among other fields.
Source link
lol
SigKAN: Signature-Weighted Kolmogorov-Arnold Networks for Time Series
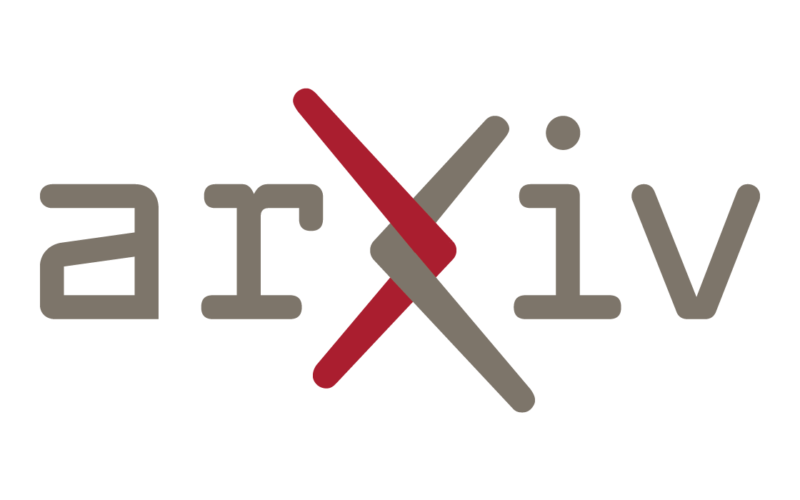