arXiv:2406.17880v1 Announce Type: new
Abstract: Video Moment Retrieval (VMR) aims to localize a specific temporal segment within an untrimmed long video given a natural language query. Existing methods often suffer from inadequate training annotations, i.e., the sentence typically matches with a fraction of the prominent video content in the foreground with limited wording diversity. This intrinsic modality imbalance leaves a considerable portion of visual information remaining unaligned with text. It confines the cross-modal alignment knowledge within the scope of a limited text corpus, thereby leading to sub-optimal visual-textual modeling and poor generalizability. By leveraging the visual-textual understanding capability of multi-modal large language models (MLLM), in this work, we take an MLLM as a video narrator to generate plausible textual descriptions of the video, thereby mitigating the modality imbalance and boosting the temporal localization. To effectively maintain temporal sensibility for localization, we design to get text narratives for each certain video timestamp and construct a structured text paragraph with time information, which is temporally aligned with the visual content. Then we perform cross-modal feature merging between the temporal-aware narratives and corresponding video temporal features to produce semantic-enhanced video representation sequences for query localization. Subsequently, we introduce a uni-modal narrative-query matching mechanism, which encourages the model to extract complementary information from contextual cohesive descriptions for improved retrieval. Extensive experiments on two benchmarks show the effectiveness and generalizability of our proposed method.
Source link
lol
MLLM as Video Narrator: Mitigating Modality Imbalance in Video Moment Retrieval
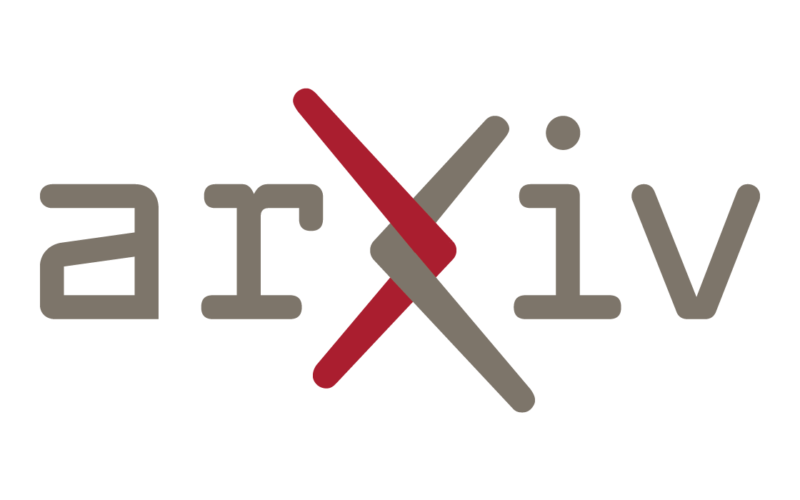