arXiv:2406.17183v1 Announce Type: new
Abstract: Novel dataset creation for all multi-object tracking, crowd-counting, and industrial-based videos is arduous and time-consuming when faced with a unique class that densely populates a video sequence. We propose a time efficient method called POPCat that exploits the multi-target and temporal features of video data to produce a semi-supervised pipeline for segmentation or box-based video annotation. The method retains the accuracy level associated with human level annotation while generating a large volume of semi-supervised annotations for greater generalization. The method capitalizes on temporal features through the use of a particle tracker to expand the domain of human-provided target points. This is done through the use of a particle tracker to reassociate the initial points to a set of images that follow the labeled frame. A YOLO model is then trained with this generated data, and then rapidly infers on the target video. Evaluations are conducted on GMOT-40, AnimalTrack, and Visdrone-2019 benchmarks. These multi-target video tracking/detection sets contain multiple similar-looking targets, camera movements, and other features that would commonly be seen in “wild” situations. We specifically choose these difficult datasets to demonstrate the efficacy of the pipeline and for comparison purposes. The method applied on GMOT-40, AnimalTrack, and Visdrone shows a margin of improvement on recall/mAP50/mAP over the best results by a value of 24.5%/9.6%/4.8%, -/43.1%/27.8%, and 7.5%/9.4%/7.5% where metrics were collected.
Source link
lol
POPCat: Propagation of particles for complex annotation tasks
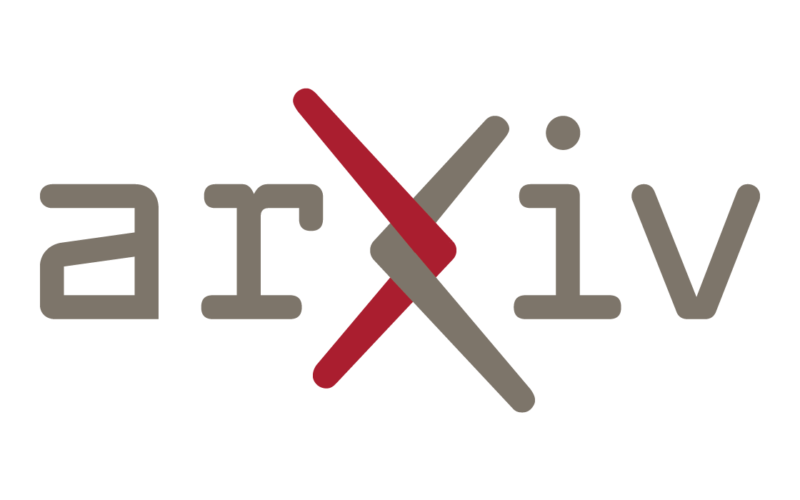