arXiv:2406.15477v1 Announce Type: new
Abstract: In the field of crisis/disaster informatics, social media is increasingly being used for improving situational awareness to inform response and relief efforts. Efficient and accurate text classification tools have been a focal area of investigation in crisis informatics. However, current methods mostly rely on single-label text classification models, which fails to capture different insights embedded in dynamic and multifaceted disaster-related social media data. This study introduces a novel approach to disaster text classification by enhancing a pre-trained Large Language Model (LLM) through instruction fine-tuning targeted for multi-label classification of disaster-related tweets. Our methodology involves creating a comprehensive instruction dataset from disaster-related tweets, which is then used to fine-tune an open-source LLM, thereby embedding it with disaster-specific knowledge. This fine-tuned model can classify multiple aspects of disaster-related information simultaneously, such as the type of event, informativeness, and involvement of human aid, significantly improving the utility of social media data for situational awareness in disasters. The results demonstrate that this approach enhances the categorization of critical information from social media posts, thereby facilitating a more effective deployment for situational awareness during emergencies. This research paves the way for more advanced, adaptable, and robust disaster management tools, leveraging the capabilities of LLMs to improve real-time situational awareness and response strategies in disaster scenarios.
Source link
lol
CrisisSense-LLM: Instruction Fine-Tuned Large Language Model for Multi-label Social Media Text Classification in Disaster Informatics
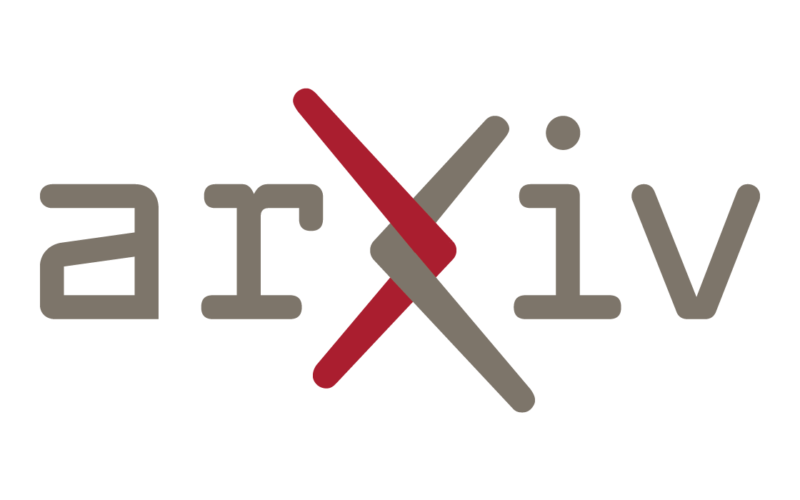