arXiv:2406.15363v1 Announce Type: new
Abstract: Large Language Models (LLMs) have demonstrated impressive and diverse abilities that can benefit various domains, such as zero and few-shot information extraction from clinical text without domain-specific training. However, for the ICD coding task, they often hallucinate key details and produce high recall but low precision results due to the high-dimensional and skewed distribution of the ICD codes. Existing LLM-based methods fail to account for the complex and dynamic interactions among the human agents involved in coding, such as patients, physicians, and coders, and they lack interpretability and reliability. In this paper, we present a novel multi-agent method for ICD coding, which mimics the real-world coding process with five agents: a patient agent, a physician agent, a coder agent, a reviewer agent, and an adjuster agent. Each agent has a specific function and uses a LLM-based model to perform it. We evaluate our method on the MIMIC-III dataset and show that our proposed multi-agent coding framework substantially improves performance on both common and rare codes compared to Zero-shot Chain of Thought (CoT) prompting and self-consistency with CoT. The ablation study confirms the proposed agent roles’ efficacy. Our method also matches the state-of-the-art ICD coding methods that require pre-training or fine-tuning, in terms of coding accuracy, rare code accuracy, and explainability.
Source link
lol
Exploring LLM Multi-Agents for ICD Coding
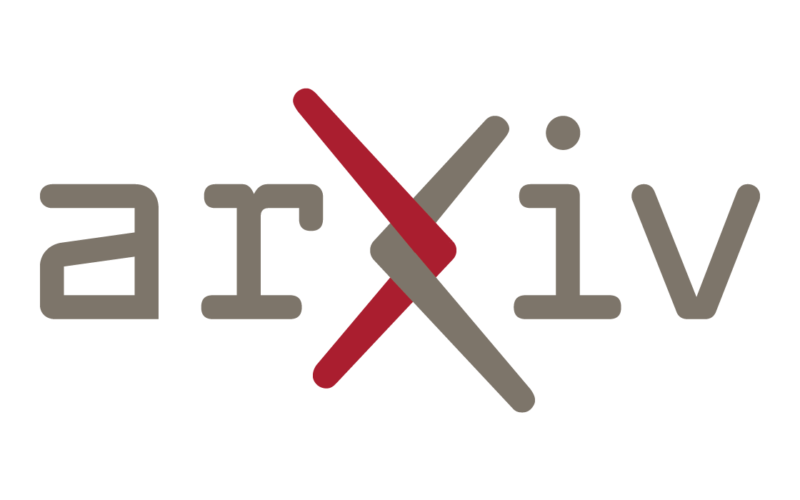