arXiv:2406.14644v1 Announce Type: new
Abstract: Data contamination has garnered increased attention in the era of large language models (LLMs) due to the reliance on extensive internet-derived training corpora. The issue of training corpus overlap with evaluation benchmarks–referred to as contamination–has been the focus of significant recent research. This body of work aims to identify contamination, understand its impacts, and explore mitigation strategies from diverse perspectives. However, comprehensive studies that provide a clear pathway from foundational concepts to advanced insights are lacking in this nascent field. Therefore, we present a comprehensive survey in the field of data contamination, laying out the key issues, methodologies, and findings to date, and highlighting areas in need of further research and development. In particular, we begin by examining the effects of data contamination across various stages and forms. We then provide a detailed analysis of current contamination detection methods, categorizing them to highlight their focus, assumptions, strengths, and limitations. We also discuss mitigation strategies, offering a clear guide for future research. This survey serves as a succinct overview of the most recent advancements in data contamination research, providing a straightforward guide for the benefit of future research endeavors.
Source link
lol
Unveiling the Spectrum of Data Contamination in Language Models: A Survey from Detection to Remediation
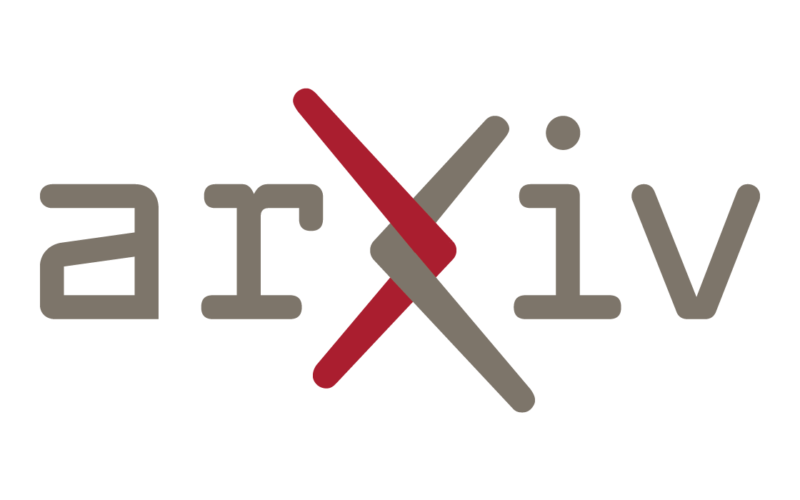