arXiv:2406.12954v1 Announce Type: new
Abstract: Skin cancer is one of the most common and deadliest types of cancer. Early diagnosis of skin cancer at a benign stage is critical to reducing cancer mortality. To detect skin cancer at an earlier stage an automated system is compulsory that can save the life of many patients. Many previous studies have addressed the problem of skin cancer diagnosis using various deep learning and transfer learning models. However, existing literature has limitations in its accuracy and time-consuming procedure. In this work, we applied five different pre-trained transfer learning approaches for binary classification of skin cancer detection at benign and malignant stages. To increase the accuracy of these models we fine-tune different layers and activation functions. We used a publicly available ISIC dataset to evaluate transfer learning approaches. For model stability, data augmentation techniques are applied to improve the randomness of the input dataset. These approaches are evaluated using different hyperparameters such as batch sizes, epochs, and optimizers. The experimental results show that the ResNet-50 model provides an accuracy of 0.935, F1-score of 0.86, and precision of 0.94.
Source link
lol
Skin Cancer Images Classification using Transfer Learning Techniques
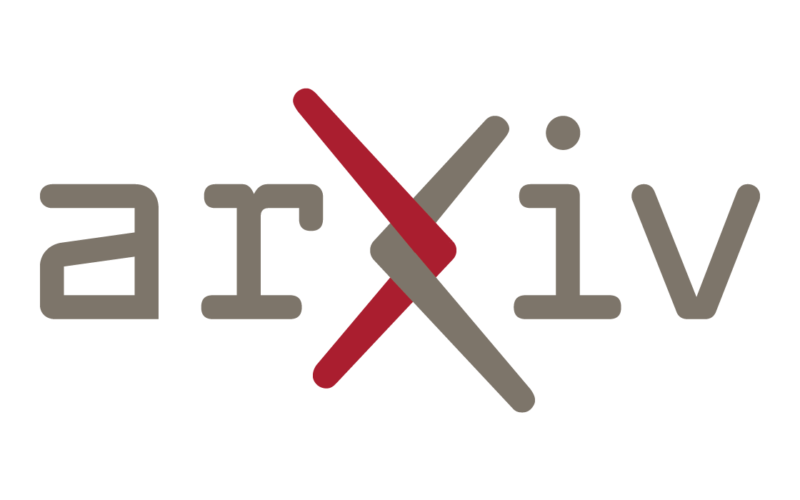