arXiv:2406.12011v1 Announce Type: new
Abstract: Recently, transductive learning methods, which leverage holdout sets during training, have gained popularity for their potential to improve speed, accuracy, and fairness in machine learning models. Despite this, the composition of the holdout set itself, particularly the balance of sensitive sub-groups, has been largely overlooked. Our experiments on CIFAR and CelebA datasets show that compositional changes in the holdout set can substantially influence fairness metrics. Imbalanced holdout sets exacerbate existing disparities, while balanced holdouts can mitigate issues introduced by imbalanced training data. These findings underline the necessity of constructing holdout sets that are both diverse and representative.
Source link
lol
The Benefits and Risks of Transductive Approaches for AI Fairness
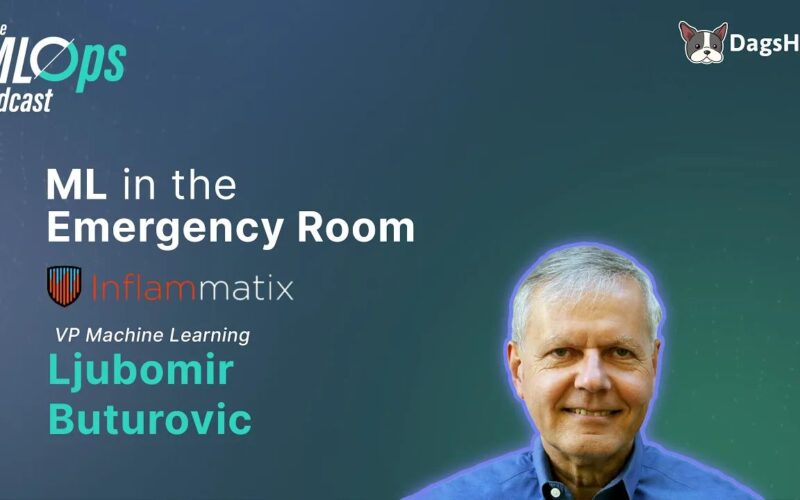