arXiv:2406.11928v1 Announce Type: new
Abstract: Multimodal electronic health record (EHR) data can offer a holistic assessment of a patient’s health status, supporting various predictive healthcare tasks. Recently, several studies have embraced the multitask learning approach in the healthcare domain, exploiting the inherent correlations among clinical tasks to predict multiple outcomes simultaneously. However, existing methods necessitate samples to possess complete labels for all tasks, which places heavy demands on the data and restricts the flexibility of the model. Meanwhile, within a multitask framework with multimodal inputs, how to comprehensively consider the information disparity among modalities and among tasks still remains a challenging problem. To tackle these issues, a unified healthcare prediction model, also named by textbf{FlexCare}, is proposed to flexibly accommodate incomplete multimodal inputs, promoting the adaption to multiple healthcare tasks. The proposed model breaks the conventional paradigm of parallel multitask prediction by decomposing it into a series of asynchronous single-task prediction. Specifically, a task-agnostic multimodal information extraction module is presented to capture decorrelated representations of diverse intra- and inter-modality patterns. Taking full account of the information disparities between different modalities and different tasks, we present a task-guided hierarchical multimodal fusion module that integrates the refined modality-level representations into an individual patient-level representation. Experimental results on multiple tasks from MIMIC-IV/MIMIC-CXR/MIMIC-NOTE datasets demonstrate the effectiveness of the proposed method. Additionally, further analysis underscores the feasibility and potential of employing such a multitask strategy in the healthcare domain. The source code is available at https://github.com/mhxu1998/FlexCare.
Source link
lol
FlexCare: Leveraging Cross-Task Synergy for Flexible Multimodal Healthcare Prediction
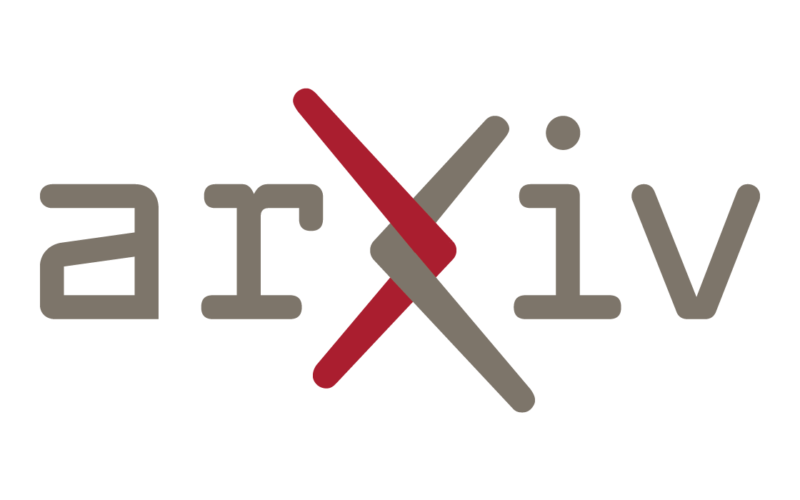