arXiv:2501.14710v1 Announce Type: cross
Abstract: Training machine learning models for fair decisions faces two key challenges: The emph{fairness-accuracy trade-off} results from enforcing fairness which weakens its predictive performance in contrast to an unconstrained model. The incompatibility of different fairness metrics poses another trade-off — also known as the emph{impossibility theorem}. Recent work identifies the bias within the observed data as a possible root cause and shows that fairness and predictive performance are in fact in accord when predictive performance is measured on unbiased data. We offer a causal explanation for these findings using the framework of the FiND (fictitious and normatively desired) world, a “fair” world, where protected attributes have no causal effects on the target variable. We show theoretically that (i) classical fairness metrics deemed to be incompatible are naturally satisfied in the FiND world, while (ii) fairness aligns with high predictive performance. We extend our analysis by suggesting how one can benefit from these theoretical insights in practice, using causal pre-processing methods that approximate the FiND world. Additionally, we propose a method for evaluating the approximation of the FiND world via pre-processing in practical use cases where we do not have access to the FiND world. In simulations and empirical studies, we demonstrate that these pre-processing methods are successful in approximating the FiND world and resolve both trade-offs. Our results provide actionable solutions for practitioners to achieve fairness and high predictive performance simultaneously.
Source link
lol
Overcoming Fairness Trade-offs via Pre-processing: A Causal Perspective
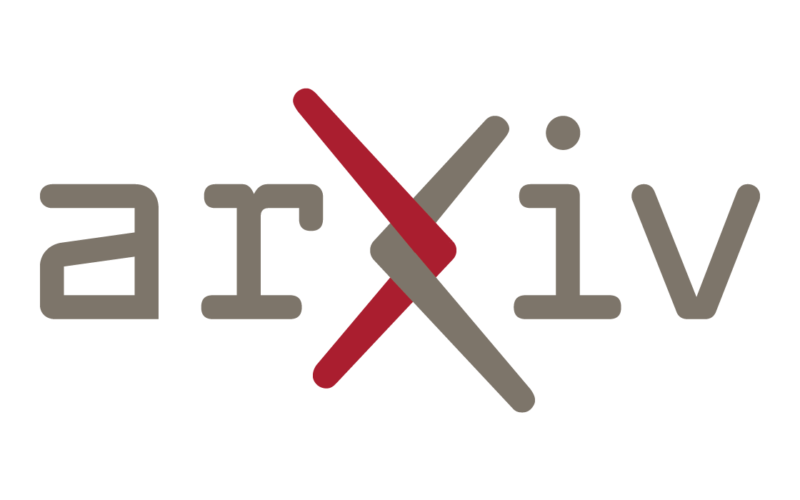