arXiv:2501.14528v1 Announce Type: new
Abstract: Idiom detection using Natural Language Processing (NLP) is the computerized process of recognizing figurative expressions within a text that convey meanings beyond the literal interpretation of the words. While idiom detection has seen significant progress across various languages, the Kurdish language faces a considerable research gap in this area despite the importance of idioms in tasks like machine translation and sentiment analysis. This study addresses idiom detection in Sorani Kurdish by approaching it as a text classification task using deep learning techniques. To tackle this, we developed a dataset containing 10,580 sentences embedding 101 Sorani Kurdish idioms across diverse contexts. Using this dataset, we developed and evaluated three deep learning models: KuBERT-based transformer sequence classification, a Recurrent Convolutional Neural Network (RCNN), and a BiLSTM model with an attention mechanism. The evaluations revealed that the transformer model, the fine-tuned BERT, consistently outperformed the others, achieving nearly 99% accuracy while the RCNN achieved 96.5% and the BiLSTM 80%. These results highlight the effectiveness of Transformer-based architectures in low-resource languages like Kurdish. This research provides a dataset, three optimized models, and insights into idiom detection, laying a foundation for advancing Kurdish NLP.
Source link
lol
Idiom Detection in Sorani Kurdish Texts
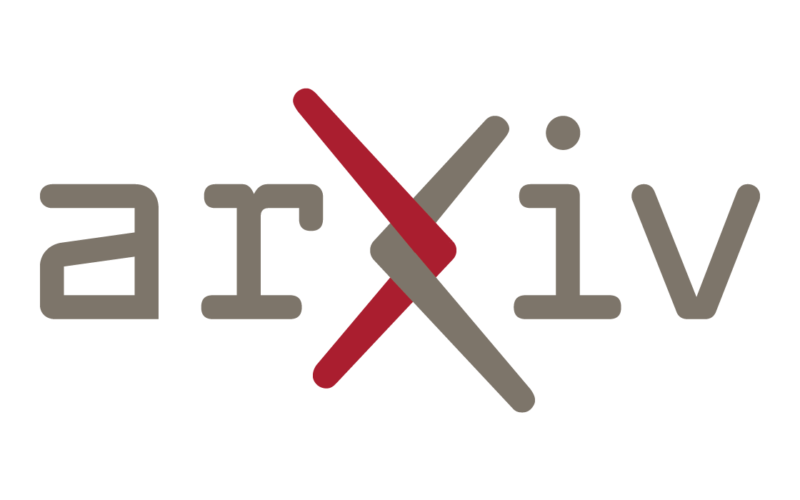