arXiv:2501.13296v1 Announce Type: new
Abstract: Importance sampling is widely used to improve the efficiency of deep neural network (DNN) training by reducing the variance of gradient estimators. However, efficiently assessing the variance reduction relative to uniform sampling remains challenging due to computational overhead. This paper proposes a method for estimating variance reduction during DNN training using only minibatches sampled under importance sampling. By leveraging the proposed method, the paper also proposes an effective minibatch size to enable automatic learning rate adjustment. An absolute metric to quantify the efficiency of importance sampling is also introduced as well as an algorithm for real-time estimation of importance scores based on moving gradient statistics. Theoretical analysis and experiments on benchmark datasets demonstrated that the proposed algorithm consistently reduces variance, improves training efficiency, and enhances model accuracy compared with current importance-sampling approaches while maintaining minimal computational overhead.
Source link
lol
Exploring Variance Reduction in Importance Sampling for Efficient DNN Training
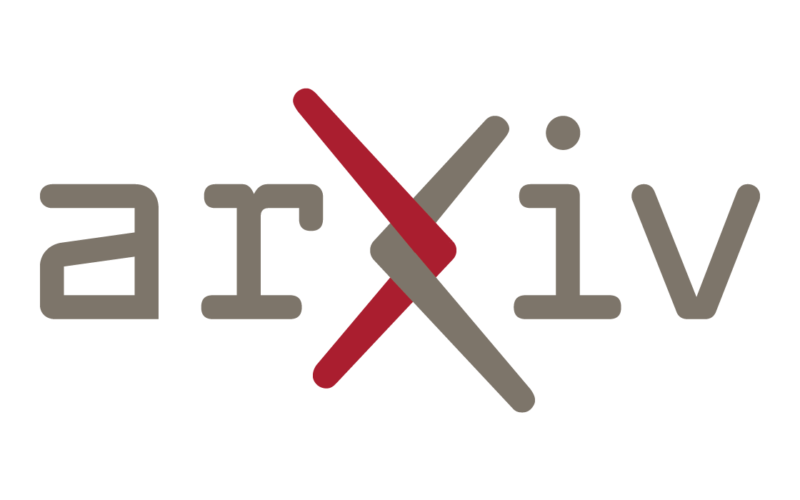