arXiv:2501.13302v1 Announce Type: new
Abstract: AI Safety Moderation (ASM) classifiers are designed to moderate content on social media platforms and to serve as guardrails that prevent Large Language Models (LLMs) from being fine-tuned on unsafe inputs. Owing to their potential for disparate impact, it is crucial to ensure that these classifiers: (1) do not unfairly classify content belonging to users from minority groups as unsafe compared to those from majority groups and (2) that their behavior remains robust and consistent across similar inputs. In this work, we thus examine the fairness and robustness of four widely-used, closed-source ASM classifiers: OpenAI Moderation API, Perspective API, Google Cloud Natural Language (GCNL) API, and Clarifai API. We assess fairness using metrics such as demographic parity and conditional statistical parity, comparing their performance against ASM models and a fair-only baseline. Additionally, we analyze robustness by testing the classifiers’ sensitivity to small and natural input perturbations. Our findings reveal potential fairness and robustness gaps, highlighting the need to mitigate these issues in future versions of these models.
Source link
lol
Watching the AI Watchdogs: A Fairness and Robustness Analysis of AI Safety Moderation Classifiers
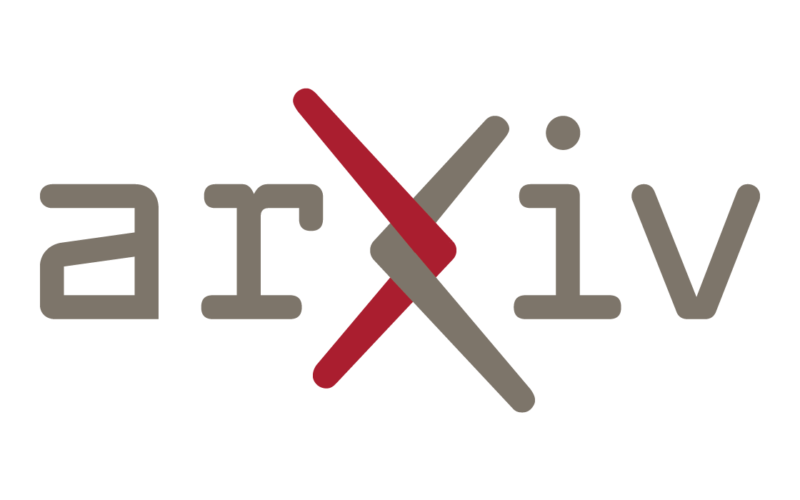