arXiv:2501.13349v1 Announce Type: new
Abstract: Diffusion-based generative models have achieved remarkable progress in visual content generation. However, traditional diffusion models directly denoise the entire image from noisy inputs, disregarding the hierarchical structure present in visual signals. This method is computationally intensive, especially for high-resolution image generation. Signal processing often leverages hierarchical decompositions; for instance, Fourier analysis decomposes signals by frequency, while wavelet analysis captures localized frequency components, reflecting both spatial and frequency information simultaneously. Inspired by these principles, we propose a multiscale diffusion framework that generates hierarchical visual representations, which are subsequently integrated to form the final output. The diffusion model target, whether raw RGB pixels or latent features from a Variational Autoencoder, s divided into multiple components that each capture distinct spatial levels. The low-resolution component contains the primary informative signal, while higher-resolution components add high-frequency details, such as texture. This approach divides image generation into two stages: producing a low-resolution base signal, followed by a high-resolution residual signal. Both stages can be effectively modeled using simpler, lightweight transformer architectures compared to full-resolution generation. This decomposition is conceptually similar to wavelet decomposition but offers a more streamlined and intuitive design. Our method, termed MSF(short for Multi-Scale Factorization), achieves an FID of 2.2 and an IS of 255.4 on the ImageNet 256×256 benchmark, reducing computational costs by 50% compared to baseline methods.
Source link
lol
MSF: Efficient Diffusion Model Via Multi-Scale Latent Factorize
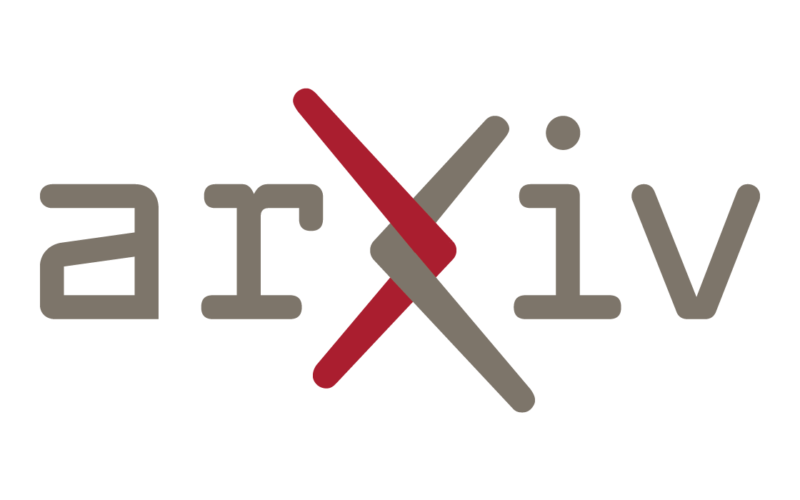