arXiv:2501.12840v1 Announce Type: new
Abstract: In clinical practice, full imaging is not always feasible, often due to complex acquisition protocols, stringent privacy regulations, or specific clinical needs. However, missing MR modalities pose significant challenges for tasks like brain tumor segmentation, especially in deep learning-based segmentation, as each modality provides complementary information crucial for improving accuracy. A promising solution is missing data imputation, where absent modalities are generated from available ones. While generative models have been widely used for this purpose, most state-of-the-art approaches are limited to single or dual target translations, lacking the adaptability to generate missing modalities based on varying input configurations. To address this, we propose an Adaptive Multi-Modality Diffusion Network (AMM-Diff), a novel diffusion-based generative model capable of handling any number of input modalities and generating the missing ones. We designed an Image-Frequency Fusion Network (IFFN) that learns a unified feature representation through a self-supervised pretext task across the full input modalities and their selected high-frequency Fourier components. The proposed diffusion model leverages this representation, encapsulating prior knowledge of the complete modalities, and combines it with an adaptive reconstruction strategy to achieve missing modality completion. Experimental results on the BraTS 2021 dataset demonstrate the effectiveness of our approach.
Source link
lol
AMM-Diff: Adaptive Multi-Modality Diffusion Network for Missing Modality Imputation
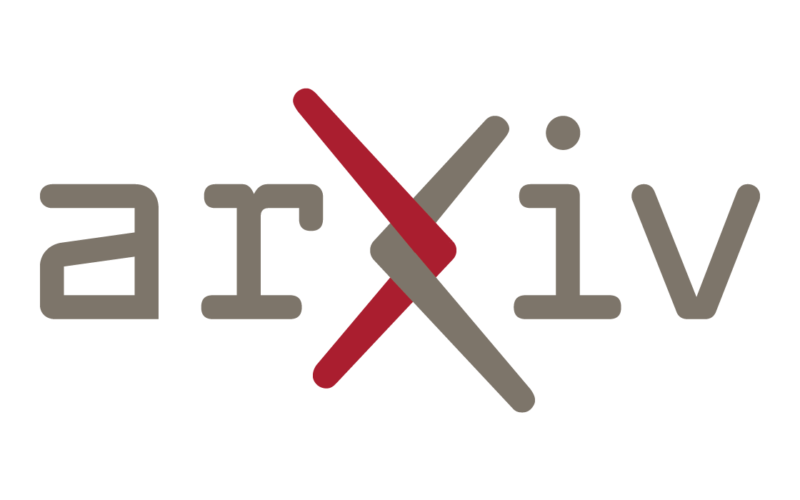