arXiv:2501.12637v1 Announce Type: new
Abstract: Neural Radiance Fields (NeRF) has achieved superior performance in novel view synthesis and 3D scene representation, but its practical applications are hindered by slow convergence and reliance on dense training views. To this end, we present DWTNeRF, a unified framework based on Instant-NGP’s fast-training hash encoding. It is coupled with regularization terms designed for few-shot NeRF, which operates on sparse training views. Our DWTNeRF includes a novel Discrete Wavelet loss that allows explicit prioritization of low frequencies directly in the training objective, reducing few-shot NeRF’s overfitting on high frequencies in earlier training stages. We additionally introduce a model-based approach, based on multi-head attention, that is compatible with INGP-based models, which are sensitive to architectural changes. On the 3-shot LLFF benchmark, DWTNeRF outperforms Vanilla NeRF by 15.07% in PSNR, 24.45% in SSIM and 36.30% in LPIPS. Our approach encourages a re-thinking of current few-shot approaches for INGP-based models.
Source link
lol
DWTNeRF: Boosting Few-shot Neural Radiance Fields via Discrete Wavelet Transform
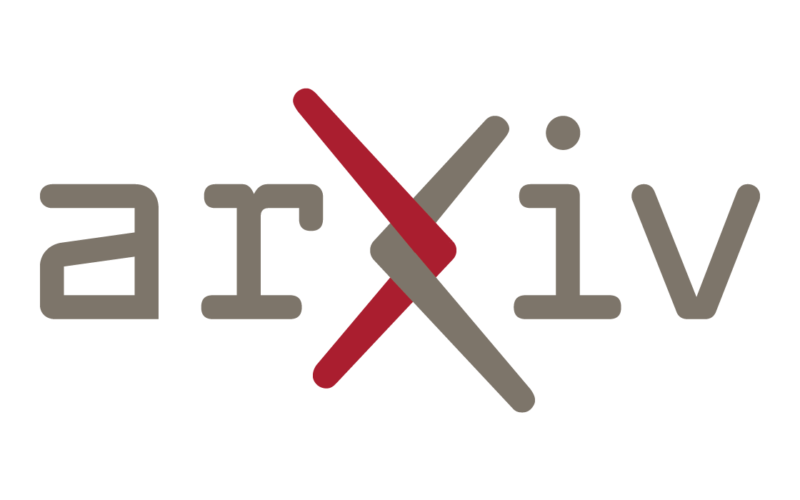