arXiv:2501.12957v1 Announce Type: cross
Abstract: We study the piecewise constant bandit problem where the expected reward is a piecewise constant function with one change point (discontinuity) across the action space $[0,1]$ and the learner’s aim is to locate the change point. Under the assumption of a fixed exploration budget, we provide the first non-asymptotic analysis of policies designed to locate abrupt changes in the mean reward function under bandit feedback. We study the problem under a large and small budget regime, and for both settings establish lower bounds on the error probability and provide algorithms with near matching upper bounds. Interestingly, our results show a separation in the complexity of the two regimes. We then propose a regime adaptive algorithm which is near optimal for both small and large budgets simultaneously. We complement our theoretical analysis with experimental results in simulated environments to support our findings.
Source link
lol
Fixed-Budget Change Point Identification in Piecewise Constant Bandits
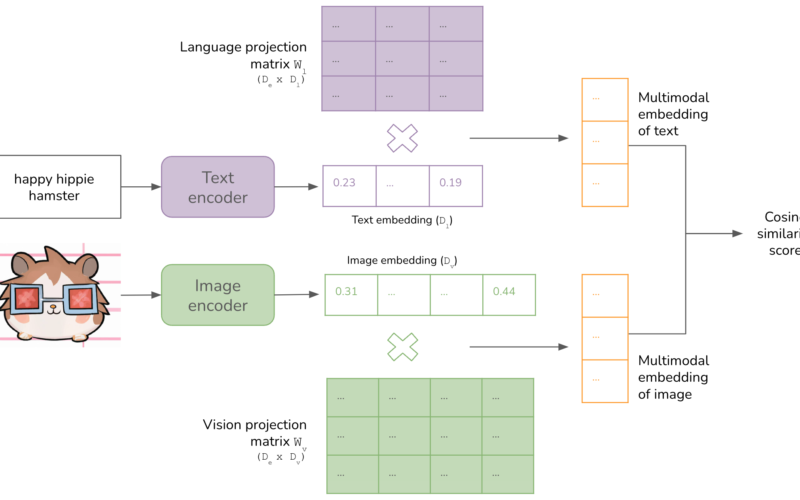