arXiv:2501.11276v1 Announce Type: cross
Abstract: Alzheimer’s disease (AD) is a common neurodegenerative disease among the elderly. Early prediction and timely intervention of its prodromal stage, mild cognitive impairment (MCI), can decrease the risk of advancing to AD. Combining information from various modalities can significantly improve predictive accuracy. However, challenges such as missing data and heterogeneity across modalities complicate multimodal learning methods as adding more modalities can worsen these issues. Current multimodal fusion techniques often fail to adapt to the complexity of medical data, hindering the ability to identify relationships between modalities. To address these challenges, we propose an innovative multimodal approach for predicting MCI conversion, focusing specifically on the issues of missing positron emission tomography (PET) data and integrating diverse medical information. The proposed incomplete triple-modal MCI conversion prediction network is tailored for this purpose. Through the missing modal generation module, we synthesize the missing PET data from the magnetic resonance imaging and extract features using specifically designed encoders. We also develop a channel aggregation module and a triple-modal co-attention fusion module to reduce feature redundancy and achieve effective multimodal data fusion. Furthermore, we design a loss function to handle missing modality issues and align cross-modal features. These components collectively harness multimodal data to boost network performance. Experimental results on the ADNI1 and ADNI2 datasets show that our method significantly surpasses existing unimodal and other multimodal models. Our code is available at https://github.com/justinhxy/ITFC.
Source link
lol
ITCFN: Incomplete Triple-Modal Co-Attention Fusion Network for Mild Cognitive Impairment Conversion Prediction
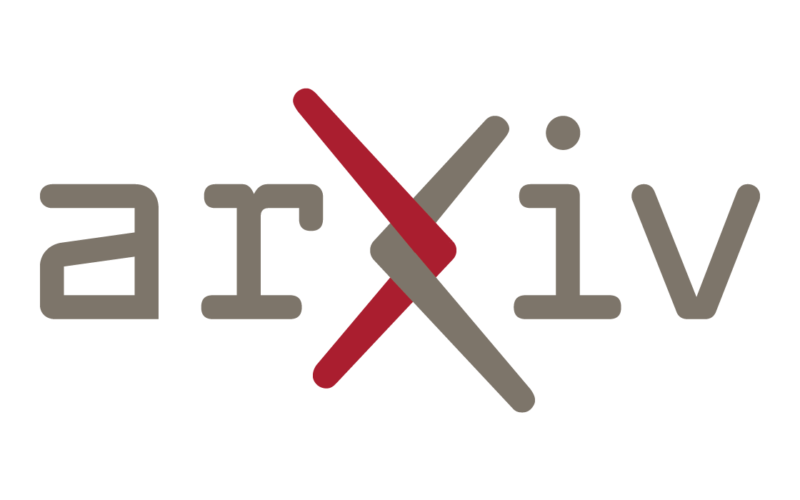