arXiv:2501.10695v1 Announce Type: new
Abstract: Conditional dependency present one of the trickiest problems in Compositional Zero-Shot Learning, leading to significant property variations of the same state (object) across different objects (states). To address this problem, existing approaches often adopt either all-to-one or one-to-one representation paradigms. However, these extremes create an imbalance in the seesaw between transferability and discriminability, favoring one at the expense of the other. Comparatively, humans are adept at analogizing and reasoning in a hierarchical clustering manner, intuitively grouping categories with similar properties to form cohesive concepts. Motivated by this, we propose Homogeneous Group Representation Learning (HGRL), a new perspective formulates state (object) representation learning as multiple homogeneous sub-group representation learning. HGRL seeks to achieve a balance between semantic transferability and discriminability by adaptively discovering and aggregating categories with shared properties, learning distributed group centers that retain group-specific discriminative features. Our method integrates three core components designed to simultaneously enhance both the visual and prompt representation capabilities of the model. Extensive experiments on three benchmark datasets validate the effectiveness of our method.
Source link
lol
Exploring Transferable Homogeneous Groups for Compositional Zero-Shot Learning
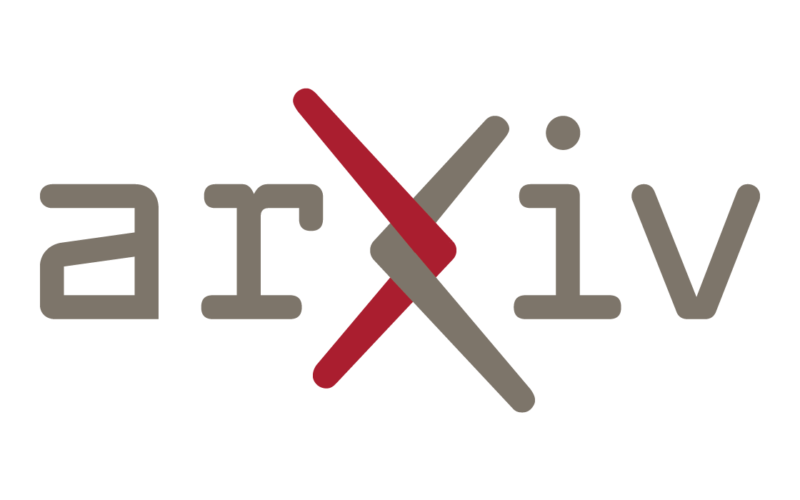