arXiv:2501.12212v1 Announce Type: cross
Abstract: Stochastic iterative algorithms, including stochastic gradient descent (SGD) and stochastic gradient Langevin dynamics (SGLD), are widely utilized for optimization and sampling in large-scale and high-dimensional problems in machine learning, statistics, and engineering. Numerous works have bounded the parameter error in, and characterized the uncertainty of, these approximations. One common approach has been to use scaling limit analyses to relate the distribution of algorithm sample paths to a continuous-time stochastic process approximation, particularly in asymptotic setups. Focusing on the univariate setting, in this paper, we build on previous work to derive non-asymptotic functional approximation error bounds between the algorithm sample paths and the Ornstein-Uhlenbeck approximation using an infinite-dimensional version of Stein’s method of exchangeable pairs. We show that this bound implies weak convergence under modest additional assumptions and leads to a bound on the error of the variance of the iterate averages of the algorithm. Furthermore, we use our main result to construct error bounds in terms of two common metrics: the L'{e}vy-Prokhorov and bounded Wasserstein distances. Our results provide a foundation for developing similar error bounds for the multivariate setting and for more sophisticated stochastic approximation algorithms.
Source link
lol
Quantitative Error Bounds for Scaling Limits of Stochastic Iterative Algorithms
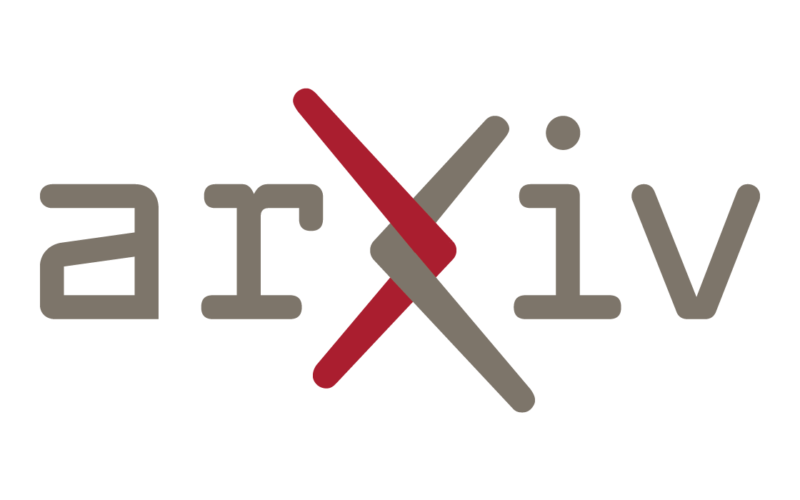