arXiv:2501.11196v1 Announce Type: cross
Abstract: Accurate and efficient segmentation of brain tumors is critical for diagnosis, treatment planning, and monitoring in clinical practice. In this study, we present an enhanced ResUNet architecture for automatic brain tumor segmentation, integrating an EfficientNetB0 encoder, a channel attention mechanism, and an Atrous Spatial Pyramid Pooling (ASPP) module. The EfficientNetB0 encoder leverages pre-trained features to improve feature extraction efficiency, while the channel attention mechanism enhances the model’s focus on tumor-relevant features. ASPP enables multiscale contextual learning, crucial for handling tumors of varying sizes and shapes. The proposed model was evaluated on two benchmark datasets: TCGA LGG and BraTS 2020. Experimental results demonstrate that our method consistently outperforms the baseline ResUNet and its EfficientNet variant, achieving Dice coefficients of 0.903 and 0.851 and HD95 scores of 9.43 and 3.54 for whole tumor and tumor core regions on the BraTS 2020 dataset, respectively. compared with state-of-the-art methods, our approach shows competitive performance, particularly in whole tumor and tumor core segmentation. These results indicate that combining a powerful encoder with attention mechanisms and ASPP can significantly enhance brain tumor segmentation performance. The proposed approach holds promise for further optimization and application in other medical image segmentation tasks.
Source link
lol
Enhancing Brain Tumor Segmentation Using Channel Attention and Transfer learning
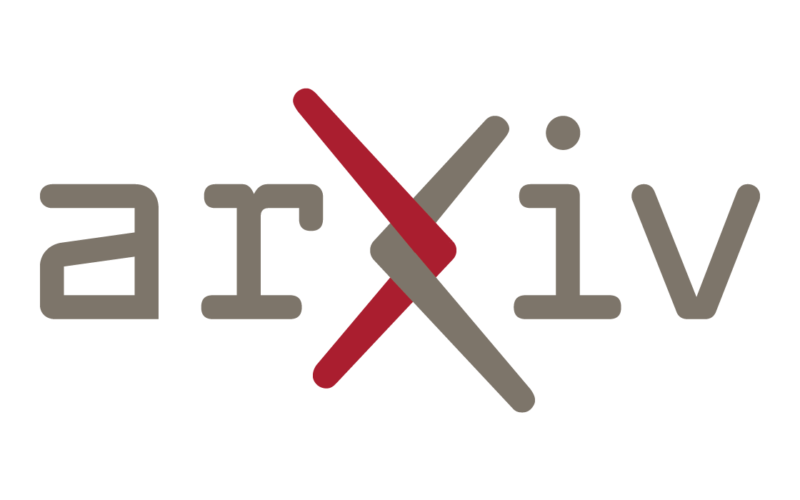